sat pronunciation go的問題,透過圖書和論文來找解法和答案更準確安心。 我們找到下列包括賽程、直播線上看和比分戰績懶人包
sat pronunciation go的問題,我們搜遍了碩博士論文和台灣出版的書籍,推薦Green, Sharon Weiner寫的 Barron’s Sat Vocabulary Flashcards 可以從中找到所需的評價。
國立臺灣大學 電機工程學研究所 李琳山所指導 王祐邦的 發音偏誤模式之督導式偵測與非督導式探勘用於電腦輔助語言學習 (2013),提出sat pronunciation go關鍵因素是什麼,來自於電腦輔助語言學習、電腦輔助發音訓練、偏誤模式偵測、偏誤模式探勘、宇集音素事後機率。
除了sat pronunciation go,大家也想知道這些:
Barron’s Sat Vocabulary Flashcards
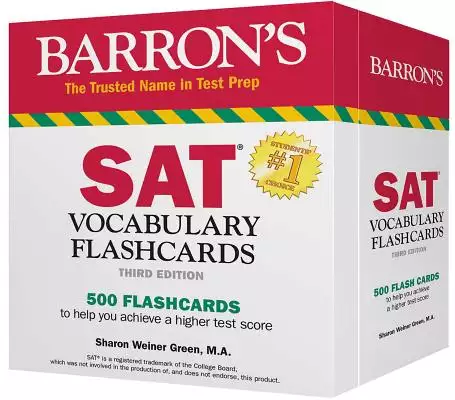
為了解決sat pronunciation go 的問題,作者Green, Sharon Weiner 這樣論述:
Boost your SAT prep with Barron's SAT Vocabulary Flashcards, which include 500 frequently tested words that have appeared in recent SAT reading passages and critical reading questions.Each flashcard includes: The target vocabulary word, along with a guide to its pronunciation and antonymsThe word's
definition, synonyms, and an example sentence that uses the wordCards are arranged alphabetically in the box, with an extra place-marker card you can use to gauge your word-learning progress. With the enclosed metal key-ring-style card holder, you can arrange cards in any order for on-the-go study s
essions. This vocabulary-building flashcard set helps students review words they might already know, as well as to master unfamiliar words they are likely to encounter both on the SAT and in their college courses.
發音偏誤模式之督導式偵測與非督導式探勘用於電腦輔助語言學習
為了解決sat pronunciation go 的問題,作者王祐邦 這樣論述:
Pronunciation error patterns (EPs) are patterns of mispronunciation frequently produced by language learners, and are usually different for different pairs of target and native languages. Accurate information of EPs can offer helpful feedbacks to the learners to improve their language skills. Howev
er, the major difficulty of EP detection comes from the fact that EPs are intrinsically similar to their corresponding canonical pronunciation, and EPs corresponding to the same canonical pronunciation are also intrinsically similar to each other. As a result, distinguishing EPs from their correspon
ding canonical pronunciation and between different EPs of the same phoneme is a difficult task – perhaps even more difficult than distinguishing between different phonemes in one language. On the other hand, the cost of deriving all EPs for each pair of target and native languages is high, usually r
equiring extensive expert knowledge or high-quality annotated data. Unsupervised EP discovery from a corpus of learner recordings would thus be an attractive addition to the field.In this dissertation, we propose new frameworks for both supervised EP detection and unsupervised EP discovery. For supe
rvised EP detection, we use hierarchical MLPs as the EP classifiers to be integrated with the baseline using HMM/GMM in a two-pass Viterbi decoding architecture. Experimental results show that the new framework enhances the power of EP diagnosis. For unsupervised EP discovery we propose the first kn
own framework, using the hierarchical agglomerative clustering (HAC) algorithm to explore sub-segmental variation within phoneme segments and produce fixed-length segment-level feature vectors in order to distinguish different EPs. We tested K-means (assuming a known number of EPs) and the Gaussian
mixture model with the minimum description length principle (estimating an unknown number of EPs) for EP discovery. Preliminary experiments offered very encouraging results, although there is still a long way to go to approach the performance of human experts. We also propose to use the universal ph
oneme posteriorgram (UPP), derived from an MLP trained on corpora of mixed languages, as frame-level features in both supervised detection and unsupervised discovery of EPs. Experimental results show that using UPP not only achieves the best performance , but also is useful in analyzing the mispronu
nciation produced by language learners.