NFL standings的問題,透過圖書和論文來找解法和答案更準確安心。 我們找到下列包括賽程、直播線上看和比分戰績懶人包
NFL standings的問題,我們搜遍了碩博士論文和台灣出版的書籍,推薦Llambes, Juan寫的 90 Years of Football Almanac: A Football Almanac With Ready References to Season Standings by Team, Coaches and Quarterbacks. 和Culpepper, Chuck的 Bloody Confused!: A Clueless American Sportswriter Seeks Solace in English Soccer都 可以從中找到所需的評價。
另外網站NFL Standings | Ponca City News也說明:Tm W L T W-L% PF PA PD MoV SoS SRS OSRS DSRS AFC East Buffalo Bills 5 1 0 .833 176 81 95 15.8 2.8 18.7 7.5 11.1 New York Jets 5 2 0 .714 159 ...
這兩本書分別來自 和所出版 。
國立臺灣科技大學 資訊工程系 范欽雄所指導 李祐任的 一個基於深度神經網路 用以預測美國職業棒球大聯盟球隊戰績的方法- 以是否晉級季後賽為例 (2020),提出NFL standings關鍵因素是什麼,來自於深度學習網路、棒球比賽、美國職棒大聯盟、球隊戰績、勝場預測、季後賽預測。
而第二篇論文國立臺灣師範大學 運動休閒與餐旅管理研究所 鄭志富所指導 翁欣瑋的 職業運動球隊社會責任、球隊認同對購買意願影響之研究-以統一7-ELEVEn獅球隊為例 (2012),提出因為有 企業社會責任、職業運動、棒球、社會認同理論的重點而找出了 NFL standings的解答。
最後網站NFL Standings in 2022/2023 Season - Football - BetInf則補充:Team % Overall Home Away 1 Buffalo Bills 83.3 5‑0‑1 (6) 2‑0‑0 (2) 3‑0‑1 (4) 2 New York Jets 71.4 5‑0‑2 (7) 1‑0‑2 (3) 4‑0‑0 (4) 3 Miami Dolphins 57.1 4‑0‑3 (7) 3‑0‑1 (4) 1‑0‑2 (3)
90 Years of Football Almanac: A Football Almanac With Ready References to Season Standings by Team, Coaches and Quarterbacks.

為了解決NFL standings 的問題,作者Llambes, Juan 這樣論述:
90 Years of Football-Almanac Is a compilation of professional football teams origins from all leagues formed in the US since 1920. Put in chronological order and in an almanac form, it provides a brief description of teams ownership and cities at the time of formation in the year they enter a pro
fessional league. The introduction provides the reader with a chronological summary by decade that describes the progress that professional football has made in since the formation of the first league APFA which became the NFL of today. The leagues section details the who's who of owners that establ
ished the leagues and teams and how and why they came about. You can follow all the teams changes in names and locations and which teams are still playing today in the PREVIOUS NAMES AND CITIES OF CURRENT TEAMS section.Follow the origin and demise of all but two of the original 14 teams to be consid
ered in the first year of the APFA/NFL in 1920, only two teams remain today, do you know who they are?See all the championship matchups, not only of teams but together with their respective coaches and quarterbacks. See all the teams with three or more consecutive appearances in a championship game
and what are the only two teams to have that distinction in the Super Bowl era (1966 to present). What team appeared in 10 consecutive championship games?The Seasons section is created to allow the reader to not only see the stats for the year of a given team but to also have the names of the coache
s and quarterbacks for that year. With this you can appreciate and see how coaches and quarterbacks move from team to team or remain with a given team year after year. You can also see how and when teams move from city to city or change ownership and names.Learn who are the for oldest teams still pl
aying the game today. How they changed names ownership and locations. Who are they today. Learn the notable events that have happened like the creation of the fourth AFL league in 1960 and then the merger with the NFL 10 years later. How the NFL addresses disparity in the number of teams merged from
the AFL, which teams change leagues and what are the leagues changed in to. How many leagues start and end after the 1970 merger of the NFL and AFL. Who starts what team and where. What coaches and quarterbacks play for these teams and then make a name for themselves in the NFL. The Almanac format
allows the reader to visualize from year to year the changes in team names, cities, coaches and quarterbacks. Also to look at teams when they were strong in a certain time frame and with which coach and quarterback at the helm.
一個基於深度神經網路 用以預測美國職業棒球大聯盟球隊戰績的方法- 以是否晉級季後賽為例
為了解決NFL standings 的問題,作者李祐任 這樣論述:
數據一直以來都出現在每個人的身邊,且與人類生活是密不可分的。近年來,數據在各領域地位日益漸增,尤其是在職業運動方面更加明顯;在所有職業運動中,棒球比賽的統計可說是數據化的先驅,例如:”Sabermetrics”是使用數據的最佳代表。棒球的數據是相對容易取得且大量的,而Major League of Baseball (MLB)又是世界上最頂級且最有名的職業棒球聯盟。本篇論文將運用深度學習的方式來預測MLB各球隊的整年度戰績區間;由於戰績預測是相對複雜且困難,而原始資料存在著大量的雜訊,導致特徵選取的重要性大大提升。我們將使用Weka做特徵的選取,再使用兩種模型來預測勝場數,且利用均方根誤差(
Root Mean Square Error; RMSE)的評斷標準跟真實勝場數做比較;此外,用預測出來的勝場數做出戰績排名表,據此,得到季後賽名單來跟實際名單做相比。本篇論文提出兩種模型來預測勝場數,其中,第一種模型,使用人工神經網路(Artificial Neural Network),而第二種模型,則會利用閘控遞迴單元網路(Gated Recurrent Unit),且資料的收集將會以2000年~2018年的數據做為訓練基礎,並以2019年的戰績作為最後的測試資料。此外,我們為了增加這些模型的信賴度,也會把2019 ZIPS球員預測成績結合2019 ZIPS 預估的球隊成績當作另一個測試
集;另外,2019 ZIPS球隊勝場預測結果,也會當成我們比較結果的標準。在最後的結果裡,人工神經網路模型表現得比閘控遞迴單元網路來的出色。接著比較把目標當成分類問題或回歸問題,當成回歸問題的結果又些許贏過視為分類問題的結果。最後比較了四種特徵選取的方式,發現關聯性方法是最好的方法。綜合上述,我們可以得到最好的模型是利用人工神經網路搭配關聯性特徵選取法來解決回歸性的問題,在利用2019真實數據當測試及測試時,並在RMSE作為評測方式下得到4.55的成績。而當使用ZIPS預估的球隊成績做為測試數據時,可得到9.04的結果。另外,在做季後賽預測測試時,可以分別得到0.93及0.73的準確率。
Bloody Confused!: A Clueless American Sportswriter Seeks Solace in English Soccer
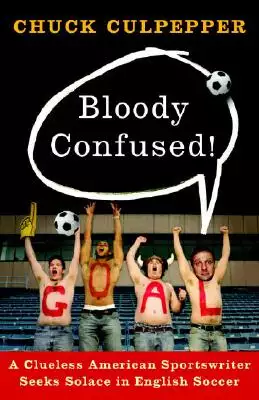
為了解決NFL standings 的問題,作者Culpepper, Chuck 這樣論述:
Chuck Culpepper was a veteran sports journalist edging toward burnout . . . then he went to London and discovered the high-octane, fanatical (and bloody confusing ) world of English soccer. After covering the American sports scene for fifteen years, Chuck Culpepper suffered from a profound case o
f Common Sportswriter Malaise. He was fed up with self-righteous proclamations, steroid scandals, and the deluge of in-your-face PR that saturated the NFL, the NBA, and MLB. Then in 2006, he moved to London and discovered a new and baffling world--the renowned Premiership soccer league. Culpepper pl
edged his loyalty to Portsmouth, a gutsy, small-market team at the bottom of the standings. As he puts it, "It was like childhood, with beer." Writing in the vein of perennial bestsellers such as Fever Pitch and Among the Thugs, Chuck Culpepper brings penetrating insight to the vibrant landscape of
English soccer--visiting such storied franchises as Manchester United, Chelsea, and Liverpool . . . and an equally celebrated assortment of pubs. Bloody Confused will put a smile on the face of any sports fan who has ever questioned what makes us love sports in the first place.
職業運動球隊社會責任、球隊認同對購買意願影響之研究-以統一7-ELEVEn獅球隊為例
為了解決NFL standings 的問題,作者翁欣瑋 這樣論述:
本研究旨在探討職業運動社會責任、球隊認同與購買意願間之關係,並選擇至現場觀看中華職棒大聯盟以臺南市立棒球場為統一7-ELEVEn獅為其主場之例行賽的統一7-ELEVEn獅隊球迷為研究對象,於2013年3月29日及30日,透過問卷調查法得有效樣本408份,以瞭解球迷在職業運動社會責任認知、球隊認同與購買意願之現況,並以描述性統計及多元逐步迴歸進行統計分析,研究發現如下:一、統一7-ELEVEn獅隊球迷的身分背景仍以年輕男性學生,月收入在一萬元以下為主要族群,大致與過往文獻相符。在接收訊息的來源上則以網路為主要媒介,顯示網路及社群網路已成為球迷在搜尋球隊資訊的重要載具。二、球團社會責任、球隊認同
與購買意願之現況皆呈正向,意味著球迷對球團在社會責任的付出上獲得球迷的肯定;球團的長期經營獲得球迷的認同;球迷對球團展現高度的支持意願。三、社會責任、球隊認同與購買意願間皆具正向解釋力,意味社會責任的投入在球隊認同的形成上已扮演關鍵之角色;球迷對社會責任認知程度的多寡影響後續消費行為;球隊認同不單單影響球迷的實際購買行為,更會影響無形的支持方式。四、社會責任與球隊認同對購買意願間具正向解釋力,且高於單一社會責任或球隊認同對購買意願之解釋力,意味球隊在具備球隊認同的基礎下,透過社會責任的落實,更能吸引球迷進場觀賽與額外消費行為之意願。 基此,本研究建議未來統一7-ELEVEn獅隊除了鞏固原
先男性學生族群外,亦可藉由主題日之舉辦以吸引不同客群進場觀賽,同時可將社會責任納入球隊運作核心,加強非賽季的社會責任活動,並將其落實在統一7-ELEVEn獅隊其他主場,以增加球隊的附加價值。
想知道NFL standings更多一定要看下面主題
NFL standings的網路口碑排行榜
-
#1.National Football League - Wikipedia
After initially determining champions through end-of-season standings, a playoff system was implemented in 1933 that culminated with the NFL Championship ... 於 en.wikipedia.org -
#2.NFL Standings Last Season - StatMuse
The Green Bay Packers finished 1st in the NFC North in 2021 with a record of 13-4. nfl standings last season. AFC. NFC. AFC East. TEAM, W, L, T, PCT, PF, PA ... 於 www.statmuse.com -
#3.NFL Standings | Ponca City News
Tm W L T W-L% PF PA PD MoV SoS SRS OSRS DSRS AFC East Buffalo Bills 5 1 0 .833 176 81 95 15.8 2.8 18.7 7.5 11.1 New York Jets 5 2 0 .714 159 ... 於 www.poncacitynews.com -
#4.NFL Standings in 2022/2023 Season - Football - BetInf
Team % Overall Home Away 1 Buffalo Bills 83.3 5‑0‑1 (6) 2‑0‑0 (2) 3‑0‑1 (4) 2 New York Jets 71.4 5‑0‑2 (7) 1‑0‑2 (3) 4‑0‑0 (4) 3 Miami Dolphins 57.1 4‑0‑3 (7) 3‑0‑1 (4) 1‑0‑2 (3) 於 www.betinf.com -
#5.Cowboys Standings | Dallas Cowboys – dallascowboys.com
AFC STANDINGS · LEGEND · LINKS · Club Links · NFL Clubs · More NFL Sites. 於 www.dallascowboys.com -
#6.2022 SEC Football Standings - Southeastern Conference
2022SEC Football news, scores, schedules, rosters, rankings, stats, live video, on-demand video. ... 2022 Standings. SEC - East ... 於 www.secsports.com -
#7.NFL Standings 2022-2023 - Scores & Odds Hub - USA Today
Get a full summary of the NFL standings. We have all the data: wins, losses and more. Stay informed with latest sports information from USA Today. 於 sportsdata.usatoday.com -
#8.NFL Standings - CBC Sports
American Football Conference. Afc East, W, L, T, PCT, PF, PA, Home, Away, DIV, Conf, STRK. Buffalo BUF, 5, 1, 0 .833, 176, 81, 2-0-0, 3-1-0, 0-1-0, 4-1-0 ... 於 stats.cbc.ca -
#9.AFC, NFC, Wild Card Regular Season Standings - NFL - TSN
Get the latest comprehensive coverage of the NFL, including a detailed look at the League, Conference and Wild-card standings. AFC East, AFC North, ... 於 www.tsn.ca -
#10.NFL Standings - NBC Sports EDGE
The NFL has suspended Chiefs EDGE Frank Clark two games for violating the personal conduct policy. This stems from his weapons arrests in 2021 per Tom Pelissero ... 於 www.nbcsportsedge.com -
#11.Standings | Chicago Bears Official Website
AFC STANDINGS · LEGEND · LINKS · Club Links · NFL Clubs · More NFL Sites. 於 www.chicagobears.com -
#12.NFL Standings - American Football - BBC Sport
Find out which american football teams are leading the pack or at the foot of the table in the NFL on BBC Sport. 於 www.bbc.co.uk -
#13.2022 NFL Standings - Pro Football Network
Year League Conference Division Team W L T PCT PF PA Net Pts Home 2022 NFL AFC AFC East Buffalo Bills 5 1 0 0.833 176 81 95 2 ‑ 0 ‑ 0 2022 NFL AFC AFC East New York Jets 5 2 0 0.714 159 137 22 1 ‑ 2 ‑ 0 2022 NFL AFC AFC East Miami Dolphins 3 3 0 0.500 131 155 ‑24 2 ‑ 1 ‑ 0 於 www.profootballnetwork.com -
#14.Football Standings - KSHSAA
SEASON STANDINGS - 2022. Scores and schedules will be kept throughout the season by each school. All schools input scores through KSHSAA website after the ... 於 www.kshsaa.org -
#15.Football Standings - TSSAAsports.com
Region 1 Region 1 Region 1 Region 1 Overall Overall Overall Overall Team W L Off Def W L Off Def Cloudland 3 0 124 64 4 5 212 295 Unaka 3 1 176 65 5 4 258 201 於 tssaasports.com -
#16.Standings - Official website of the New England Patriots
NFC STANDINGS · LEGEND · LINKS · Club Links · NFL Clubs · More NFL Sites. 於 www.patriots.com -
#17.NFL Standings Archives - NESN.com
NFL Standings ; AFC West · Kansas CityKC ; AFC East · BuffaloBUF ; AFC South · TennesseeTEN ; AFC North · BaltimoreBAL ... 於 nesn.com -
#18.Football Standings - Pac-12
Official Pac-12 Conference football standings. ... Only one win against a team from the NCAA Football Championship Subdivision or lower division will be ... 於 pac-12.com -
#19.2021 Football Standings - Patriot League
School PL PCT Overall Overall PCT Home Away Neutral Streak Holy Cross 6‑0 1.000 10‑3.769 4‑2 6‑1 0‑0 L1 Colgate 5‑1.833 5‑6.455 3‑2 2‑4 0‑0 W3 Fordham 4‑2.667 6‑5.545 3‑2 3‑3 0‑0 L2 於 patriotleague.org -
#20.Football: NFL 2022/2023 Standings - Livesport.com
NFL standings, NFL 2022/2023 tables. Find overall/home/away standings, results and fixtures. 於 www.livesport.com -
#21.NFL 2022/2023 Standings - American football/USA
Follow NFL 2022/2023 standings, overall, home/away and form (last 5 games) NFL 2022/2023 standings. 於 www.flashscore.com -
#22.Ellis County high school football standings
Ellis County high school football standings. October 26, 2022. in Sports · Facebook Twitter Email WhatsApp Share. UIL District 11-6A ;Dist;All;PF;PA ... 於 www.waxahachietx.com -
#23.NFL Standings | NFL Information | IBD - Insiders Betting Digest
Find all about the updated NFL Standings 2022 for this season & check out the NFL season predictions at Insiders Betting Digest. 於 insidersbettingdigest.com -
#24.2022 NFL Football Standings - CBSSports.com
East W Wins L Losses T Ties PCT Win Percentage PF Points for PA Points allowed Buffalo 5 1 0.833 176 81 N.Y. Jets 5 2 0.714 159 137 Miami 4 3 0.571 147 165 於 www.cbssports.com -
#25.Dustin Baker's 2022 NFL Standings Predictions
Dustin Baker's 2022 NFL Standings Predictions · Buffalo Bills: 13-4. Miami Dolphins: 9-8 · Baltimore Ravens: 11-6 **Cincinnati Bengals: 10-7 於 vikingsterritory.com -
#26.2021 NFL Standings - The Boston Globe
Get the latest NFL football standings. Follow your favorite team and find 2021 team wins and losses, home and away, records, current streak, ... 於 www.bostonglobe.com -
#27.NFL Standings | National Post
Oct 28 (Stats Perform) – Standings from the NFL games on Thursday. 於 nationalpost.com -
#28.NFL Standings - Vizlib
The Vizlib Data Viz Health Check Get your free Data Viz fitness score! Close. Vizlib. Products. Vizlib Library ... 於 home.vizlib.com -
#29.Standings - Jacksonville Jaguars
Jacksonville Jaguars Standings: The official source of the current Jaguars season standings within the division. ... NFL Clubs. NFL Clubs. 於 www.jaguars.com -
#30.NFL standings 2022: Kansas City Chiefs reign supreme in ...
Recent Super Bowl winners · 2022: Los Angeles Rams over Cincinnati Bengals (23-20) · 2021: Tampa Bay Buccaneers over Kansas City Chiefs (31-9) ... 於 sportsnaut.com -
#31.The NFL Standings Are a Beautiful Mess - The Ringer
The NFL's best teams aren't as good this season as they were 2015, and the worst teams aren't as bad. Coming into this week's games, there were ... 於 www.theringer.com -
#32.2022 GAC FOOTBALL STANDINGS
Team School GAC Conf GAC Pct Overall Overall Pct Home Away Ne... Ouachita Baptist Ouachita Baptist 9‑0 9‑0 1.000 9‑0 1.000 5‑0 3‑0 1‑0 East Central East Central 6‑3 6‑3.667 6‑3.667 3‑1 3‑2 0‑0 Harding Harding 6‑2 6‑2.750 6‑2.750 3‑1 3‑1 0‑0 於 greatamericanconference.com -
#33.Broncos Standings | Denver Broncos – denverbroncos.com
NFC STANDINGS · LEGEND · LINKS · Club Links · NFL Clubs · More NFL Sites. 於 www.denverbroncos.com -
#34.2021 NFL standings after Week 3: Chiefs, Steelers, Seahawks ...
Checking in on the 2021 NFL standings after Week 3 as some shocking teams are in last place in their division, including the Chiefs and ... 於 nflspinzone.com -
#35.Breaking down the NFL standings coming out of Week 7
Below are the eight divisional standings, along with some quick thoughts about each division after seventh weeks of football. We'll update as ... 於 dknation.draftkings.com -
#36.2022 Football Standings - Sun Belt Conference
The official 2022 Football Standings for Sun Belt Conference. 於 sunbeltsports.org -
#37.NFL Football - Standings & Betting Records | Odds Shark
NFL Football Standings - Including Against the Spread, Home & Away Record and Handicapping Data. 於 www.oddsshark.com -
#38.NFL Standings: Week 17 playoff picture after Packers beat ...
NFL Standings : Week 17 playoff picture after Packers beat Vikings to clinch No. 1 seed in NFC · 1. Green Bay Packers (13-3, clinched NFC North ... 於 www.nj.com -
#39.1933 NFL Standings & Team Stats | Pro-Football-Reference.com
Check out the 1933 NFL Standings & Team Stats including AFC and NFC results and standings on Pro-football-reference.com. 於 www.pro-football-reference.com -
#40.NFL News, Standings, Games & Results - American Football
NFL - National Football League news from Sky Sports. 於 www.skysports.com -
#41.NFL Standings 2022 - ESPN
Visit ESPN to view the 2022 NFL Standings. ... Standings · Playoff · Expanded · Vs. Division · LeagueConferenceDivision. American Football Conference ... 於 www.espn.com -
#42.NFL Division Standings | FOX Sports
Visit FOXSports.com for NFL Division standings, conference rankings, updated NFL records and playoff standings. Filter by conference, division, ... 於 www.foxsports.com -
#43.NFL Standings - Sportsnet.ca
Your source for up to date NFL regular season standings. 於 www.sportsnet.ca -
#44.2022 Football Standings
The official 2022 Football Standings for Heartland Collegiate Athletic Conference. 於 heartlandconf.org -
#45.2022-23 NFL Football Standings - Full AFC and NFC ...
Breaking NFL news and in-depth analysis from the best newsroom in sports. Follow your favorite teams. ... NFL Standings. Wild Card Division Conference ... 於 theathletic.com -
#46.Football | NFL | Standings - The Washington Post
NFL Standings. Division | Conference. American Football Conference. East Division. W, L, T, Pct ... 於 stats.washingtonpost.com -
#47.NFL division and wild card standings | AJC Sports
NFL Standings. Today's Games | Atlanta Falcons Schedule | Standings | Depth Chart | Player Stats | Team Stats | Injuries. Standings. 2022. 於 www.ajc.com -
#48.Standings - Sports - MSN
Standings are not available yet. Check back later. News. Look: Tom Brady Made Unfortunate NFL History Tonight. The Spun. Fansided. 於 www.msn.com -
#49.Ravens Standings | Baltimore Ravens – baltimoreravens.com
NFC STANDINGS · LEGEND · LINKS · Club Links · NFL Clubs · More NFL Sites. 於 www.baltimoreravens.com -
#50.Updated NFC Playoff Picture, NFC Wild Card standings after ...
NFC Wild Card standings after Thursday Night Football Week 8 · New York Giants (6-1) · Dallas Cowboys (5-2) · Los Angeles Rams (3-3) · Sean ... 於 fansided.com -
#51.2022 Football Standings - American Athletic Conference
School Conf Overall CPct Pct Home Away Neutral Streak Tulane 4‑0 7‑1 1.000.875 4‑1 3‑0 0‑0 W4 Cincinnati 3‑0 6‑1 1.000.857 3‑0 2‑1 1‑0 W6 UCF 2‑1 5‑2.667.714 4‑1 1‑1 0‑0 L1 於 theamerican.org -
#52.NFL Standings 2022 | Football Standings & Records - Lines.com
Get up-to-date NFL football standings from around the league. See the updated and revised schedule for the 2022-2023 season. 於 www.lines.com -
#53.NFL Standings - - NYTimes.com
NFL Standings. Division | Conference. American Football Conference. East Division. W, L, T, Pct ... 於 nytimes.stats.com -
#54.Browns Standings | Cleveland Browns – clevelandbrowns.com
NFC STANDINGS · LEGEND · LINKS · Club Links · NFL Clubs · More NFL Sites. 於 www.clevelandbrowns.com -
#55.Building the NFL Standings Table • reactablefmtr - GitHub Pages
2021 NFL Standings & Team Ratings. . Team Scoring & Margin of Victory. Team Rating (SRS). 於 kcuilla.github.io -
#56.Standings - Philadelphia Eagles
AFC STANDINGS · LEGEND · LINKS · Club Links · NFL Clubs · More NFL Sites. 於 www.philadelphiaeagles.com -
#57.2022 NFL Division Standings
See the latest NFL Standings by Division, Conference and League. Find current or past season NFL standings by team. 於 www.nfl.com -
#58.NFL Standings Schedule, Scores & Stats | NBC Sports
Find the latest NFL Standings by Division, Conference and Team. Stay up to date with the NFL season standings on NBC Sports. 於 scores.nbcsports.com -
#59.NFL Team Standings & Playoff Picture | SIA Insights
NFL standings change week-by-week as the playoffs approach. Whether you're looking for the latest information on conference and division standings, ... 於 news.sportsinteraction.com -
#60.Chargers Standings | Los Angeles Chargers - chargers.com
NFC STANDINGS · LEGEND · LINKS · Club Links · NFL Clubs · More NFL Sites. 於 www.chargers.com -
#61.2022-2023 6A Football Standings | AZPreps365
6A Standings. Football. 6A · 5A · 4A · 3A · 2A · 1A; 2022-2023. 2022-2023 · 2021-2022 · 2020-2021 · 2019-2020 · 2018-2019 · 2017-2018 · 2016-2017 ... 於 azpreps365.com -
#62.NFL Football Standings
STANDINGS | PLAYOFF PICTURE | POWER RANKINGS. As of Tuesday, October 25, 2022 12:34:20 AM EDT. American Football Conference ... 於 newsday.sportsdirectinc.com -
#63.NFL Standings - Picksfootball.com
AFC EAST W L T AVG PF PA NP H A Bills 5 1 0.833 176 81 95 2‑0 3‑1 Jets 5 2 0.714 159 137 22 1‑2 4‑0 Dolphins 4 3 0.571 147 165 ‑18 3‑1 1‑2 於 www.picksfootball.com -
#64.2022 Football Standings - Atlantic Coast Conference
School ACC Overall Pct Pct Home Away Neutral Division Streak Clemson 6‑0 8‑0 1.000 1.000 4‑0 4‑0 0‑0 5‑0 W8 Syracuse 3‑1 6‑1.750.857 5‑0 1‑1 0‑0 2‑1 L1 Wake Forest 2‑1 6‑1.667.857 4‑1 2‑0 0‑0 2‑1 W3 於 theacc.com -
#65.Standings - Seattle Seahawks
Seattle Seahawks Standings: The official source of the current Seahawks season standings in the NFC West and all standings of the NFL. 於 www.seahawks.com -
#66.NFL Standings by Division 2022/23 - Marca
Get all the latest updates on the NFL 2022/2023 Standings by Division on Marca. Full NFC and AFC Standings and game stats. 於 www.marca.com -
#67.NFL Standings 2022-23 - Football Standings - Vegas Insider
Keep track of the NFL Standings during the 2023-23 Football season from week 1 to week 18 and discover division records and AFC & NFC ... 於 www.vegasinsider.com -
#68.NFL Standings - Newsday - Gracenote
American Football Conference. Afc East, W, L, T, PCT, PF, PA, Home, Away, DIV, Conf, STRK. Buffalo BUF, 5, 1, 0 .833, 176, 81, 2-0-0, 3-1-0, 0-1-0, 4-1-0 ... 於 newsday.sports.gracenote.com -
#69.2022 Football Standings
The official 2022 Football Standings for Northern Sun Intercollegiate Conference. 於 northernsun.org -
#70.2022 National Football League standings by ... - AS USA
Find out the updated National Football League football standings, stats, and scores by Division, Conference and League. Check the record, rankings and ... 於 en.as.com -
#71.Nfl Standings - Etsy
Check out our nfl standings selection for the very best in unique or custom, handmade pieces from our wall hangings shops. 於 www.etsy.com -
#72.NFL Standings By Division, League - ClutchPoints
NFL standings organized by division (AFC North, AFC East, AFC South, AFC West, NFC North, NFC East, NFC South, NFC West), league (AFC, NFC) 於 clutchpoints.com -
#73.NFL Standings - Boston Sports Journal
Scores. NFL · NHL · MLB · Logo · Standings · Statistics · Schedule. NFL. AFC. East. W. L. T. PCT. PF. PA. Div. Conf. Strk. Dolphins, 3, 1, 0 .750, 98, 91 ... 於 www.bostonsportsjournal.com -
#74.NFL Standings - Scores and Stats
Get NFL Standings by division. See who leads each National Football League division here at ScoresAndStats.com. 於 www.scoresandstats.com -
#75.Colin Cowherd Predicts the Entire NFL Standings For the ...
Colin Cowherd predicts the NFL standings for the entire league with training camps set to begin next week, as Colin names his four division ... 於 foxsportsradio.iheart.com -
#76.The 2021 NFL standings as predicted by oddsmakers | Offside
Predicting the 2021 NFL standings · Dallas Cowboys (9) · Washington Football Team (8.5) · New York Giants (7) · Philadelphia Eagles (6.5). 於 dailyhive.com -
#77.Bengals Standings | Cincinnati Bengals – bengals.com
NFC STANDINGS · LEGEND · LINKS · Club Links · NFL Clubs · More NFL Sites. 於 www.bengals.com -
#78.NFL Standings - FantasyData
NFL standings for current and past seasons. FantasyData.com also provides real time NFL statistics and a historical football database. 於 fantasydata.com -
#79.2022 NFL Standings | The Football Database
The following are the current 2022 NFL Standings. x - Clinched Playoff Spot, y - Clinched Division, z - Clinched Home Field. NFL. 於 www.footballdb.com -
#80.NFL Standings on Yahoo Sports
Comprehensive National Football League news, scores, standings, fantasy games, rumors, and more. 於 sports.yahoo.com -
#81.NFL Standings 2022 - Lineups
Live NFL Standings for all NFL teams plus standings analysis. Sortable with recent history stats. Click through for full team stats, schedule and rosters. 於 www.lineups.com -
#82.Football - Standings - Eastern Cincinnati Conference
Conference Conference Conference Conference OVERALL OVERALL OV... TEAMS W‑L PCT PF PA W‑L PCT PF Winton Woods 9 ‑ 0 1.000 388 110 10 ‑ 0 1.000 427 Kings 8 ‑ 1.889 338 115 9 ‑ 1.900 372 於 eccsports.com -
#83.NFL Results And Standings | Barron's
Standings (w l t pct pf pa). American Football Conference. East. Buffalo 5 1 0 .833 176 81. N.Y. Jets 5 2 0 .714 159 137. 於 www.barrons.com -
#84.NFL Standings, Week 4: Chiefs maintain AFC West lead
After the conclusion of Week 4 in the NFL season, what were the outcomes and where do the KC Chiefs stand in the AFC West and AFC standings? 於 arrowheadaddict.com -
#85.Updated Mid-Penn Conference football standings through Oct ...
Updated Mid-Penn Conference football standings through Oct. 25. Published: Oct. 26, 2022, 6:00 a.m.. CD East scrimmages Bishop McDevitt in high school ... 於 www.pennlive.com -
#86.NFL Standings - JT-SW.com
NFL Historical · Team Histories · Games · Annual Stats · Player/Coach Stats · Rosters · Box Scores. NFL Standings ... 於 www.jt-sw.com -
#87.NFL Standings - Sporting News
NFL Standings ; AFC East · Bills, 5 ; AFC North · Ravens, 4 ; AFC South · Titans, 4 ; AFC West · Chiefs, 5 ; NFC East · Eagles, 6 ... 於 www.sportingnews.com -
#88.NFL Standings Predictions for the 2022 Season - YouTube
In this video, I predict every NFL team's recording for the upcoming 2022 season! Is there anything you would change with this prediction? 於 www.youtube.com -
#89.NFL Football Standings Projections on TeamRankings.com
NFL Standings Projections. AFC. Current, Projection, Playoffs. AFC East, W, L, T, W ... 於 www.teamrankings.com -
#90.Brazilian football standings - Xinhua
Brazilian football standings- ... RIO DE JANEIRO, Oct. 27 (Xinhua) -- Following are the standings after Thursday's matches in Brazil's Serie ... 於 english.news.cn -
#91.What will the standings look like for the 2022 NFL season?
What will the standings look like for the 2022 NFL season? How will the Rams fare in defending their Super Bowl crown? 於 www.turfshowtimes.com -
#92.nfl standings board - Amazon.com
Price and other details may vary based on product size and color. Party Animal NHL Magnetic Standings Board, Includes 32 NHL Team Magnets. 於 www.amazon.com -
#93.Cute NFL Standings Tracker Uses Little Mini Helmets
Cute NFL Standings Tracker Uses Little Mini Helmets ... The tracker displays the NFL league table with a ten-minute delay, thanks to a paid ... 於 hackaday.com -
#94.NFL Standings - HomeTeamsONLINE
Team, Record, Win %, Home, Away, PF, PA, Streak. AFC East. Patriots, 0-0, 0-0, 0-0, 0, 0. Jets, 0-0, 0-0, 0-0, 0, 0. Dolphins, 0-0, 0-0, 0-0, 0, 0. 於 www.hometeamsonline.com -
#95.NFL Standings | Tampa Bay Buccaneers
Tampa Bay Buccaneers Standings: The official source of the current Buccaneers season standings within the division. 於 www.buccaneers.com -
#96.NFL Standings and Betting Records 2022-2023 - Covers.com
AFC East W L T PF PA Home Away Conf Non Conf Div ATS O/U Buffalo 5 1 0 176 81 2‑0‑0 3‑1‑0 4‑1‑0 1‑0‑0 0‑1‑0 4‑1‑1 1‑5‑0 N.Y. Jets 5 2 0 159 137 1‑2‑0 4‑0‑0 4‑2‑0 1‑0‑0 1‑0‑0 5‑2‑0 3‑4‑0 Miami 4 3 0 147 165 3‑1‑0 1‑2‑0 4‑2‑0 0‑1‑0 2‑1‑0 3‑4‑0 2‑5‑0 於 www.covers.com -
#97.NFL Standings - Sports Illustrated
Visit SI.com for the latest NFL standings, wild card standings, and current playoff picture. 於 www.si.com -
#98.NFL Standings 2021-22: Week 16 Records, Playoff Scenarios ...
Before those matchups take place, here's a look at the current NFL standings and the playoff landscape for both the AFC and NFC. 於 bleacherreport.com -
#99.NFL : standings - pick a year - mcubed.net
Support a small business and visit Fools Fairy. Great gifts for your wife/gf. Home · NFL · NFL teams Super Bowls Series Records Standings ... 於 mcubed.net