Oncology的問題,我們搜遍了碩博士論文和台灣出版的書籍,推薦Beriwal, Sushil,Huq, M. Saiful,Boyiadzis, Michael M.寫的 Radiation-Oncology Therapy 和Varadhan, Ravi,Zhou, Hua的 Acceleration of the Em, MM, and Other Monotone Algorithms for Modern Applications都 可以從中找到所需的評價。
另外網站Oncology - Therapeutic Focus Areas - Our Science | AbbVie也說明:AbbVie in Oncology: Committed to transforming standards of care. We continue to advance our dynamic pipeline to deliver new innovations for cancer patients.
這兩本書分別來自 和所出版 。
國立臺北科技大學 電資學院外國學生專班(iEECS) 白敦文所指導 VAIBHAV KUMAR SUNKARIA的 An Integrated Approach For Uncovering Novel DNA Methylation Biomarkers For Non-small Cell Lung Carcinoma (2022),提出Oncology關鍵因素是什麼,來自於Lung Cancer、LUAD、LUSC、NSCLC、DNA methylation、Comorbidity Disease、Biomarkers、SCT、FOXD3、TRIM58、TAC1。
而第二篇論文國防醫學院 醫學科學研究所 高啟雯所指導 謝慧玲的 以疾病不確定感理論發展整合性心動健康網路照顧模式提升心房顫動病人因應策略之成效探討 (2021),提出因為有 整合性照顧、移動健康醫療、心房顫動、疾病不確定感、因應策略的重點而找出了 Oncology的解答。
最後網站Labcorp Oncology: Diagnostic Testing for Cancer Treatment則補充:Labcorp Oncology provides cancer testing services and genetic testing for cancer predisposition.
Radiation-Oncology Therapy
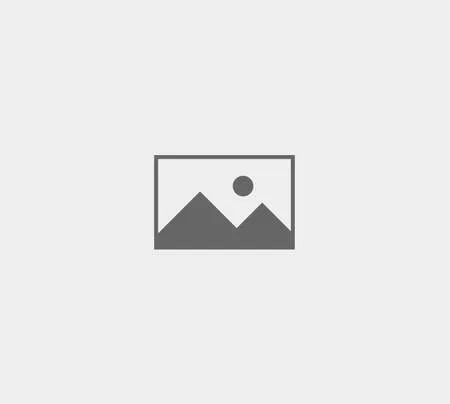
為了解決Oncology 的問題,作者Beriwal, Sushil,Huq, M. Saiful,Boyiadzis, Michael M. 這樣論述:
Sushil Beriwal, MD, is Professor of Radiation Oncology and Director, Residency, Department of Radiation Oncology, University of Pittsburgh School of Medicine. Mohammed Saiful Huq, PhD, is Professor Radiation Oncology, Director of Medical Physics Division, Department of Radiation Oncology, Universi
ty of Pittsburgh School of Medicine.Michael Boyiadzis, MD, M.H.Sc, is Associate Professor of Medicine and Translational Science, Division of Hematology-Oncology, University of Pittsburgh School of Medicine, UPMC Hillman Cancer Center.
Oncology進入發燒排行的影片
甲狀腺癌 - 陳穎樂臨床腫瘤科專科醫生@FindDoc.com
FindDoc Facebook : https://www.facebook.com/FindDoc
FindDoc WeChat : 快徳健康香港 FindDoc
FindDoc Instagram:@finddochk
(一)甲狀腺癌有什麼類型? 00:06
(二)甲狀腺癌有什麼治療方法? 00:42
(三)患者有機會對放射治療無反應嗎? 01:45
(四)何時展開標靶藥物治療可得到最佳效果呢? 03:00
(本短片作健康教育之用,並不可取代任何醫療診斷或治療。治療成效因人而異,如有疑問,請向專業醫療人士諮詢。)
參考資料:
1. 香港癌症資料統計中心. (2020). 2018 年香港癌症統計概覽. Retrieved from https://www3.ha.org.hk/cancereg/pdf/overview/Overview%20of%20HK%20Cancer%20Stat%202018_tc.pdf
2. 醫院管理局. (2020). 甲狀腺癌. Retrieved from https://www21.ha.org.hk/smartpatient/SPW/zh-hk/Disease-Information/Disease/?guid=1163a3df-86db-4b3b-a325-45741fcb04be
3. Cabanillas, M. E., & Habra, M. A. (2016). Lenvatinib: Role in thyroid cancer and other solid tumors. Cancer treatment reviews, 42, 47–55. https://doi.org/10.1016/j.ctrv.2015.11.003
4. Cabanillas, M. E., & Takahashi, S. (2019). Managing the adverse events associated with lenvatinib therapy in radioiodine-refractory differentiated thyroid cancer. Seminars in oncology, 46(1), 57–64. https://doi.org/10.1053/j.seminoncol.2018.11.004
5. Sugino K, et al. Endocr J 2018;299-306
資料來源:https://www.FindDoc.com
查詢醫生資訊:
https://www.finddoc.com
An Integrated Approach For Uncovering Novel DNA Methylation Biomarkers For Non-small Cell Lung Carcinoma
為了解決Oncology 的問題,作者VAIBHAV KUMAR SUNKARIA 這樣論述:
Introduction - Lung cancer is one of primal and ubiquitous cause of cancer related fatalities in the world. Leading cause of these fatalities is non-small cell lung cancer (NSCLC) with a proportion of 85%. The major subtypes of NSCLC are Lung Adenocarcinoma (LUAD) and Lung Small Cell Carcinoma (LUS
C). Early-stage surgical detection and removal of tumor offers a favorable prognosis and better survival rates. However, a major portion of 75% subjects have stage III/IV at the time of diagnosis and despite advanced major developments in oncology survival rates remain poor. Carcinogens produce wide
spread DNA methylation changes within cells. These changes are characterized by globally hyper or hypo methylated regions around CpG islands, many of these changes occur early in tumorigenesis and are highly prevalent across a tumor type.Structure - This research work took advantage of publicly avai
lable methylation profiling resources and relevant comorbidities for lung cancer patients extracted from meta-analysis of scientific review and journal available at PubMed and CNKI search which were combined systematically to explore effective DNA methylation markers for NSCLC. We also tried to iden
tify common CpG loci between Caucasian, Black and Asian racial groups for identifying ubiquitous candidate genes thoroughly. Statistical analysis and GO ontology were also conducted to explore associated novel biomarkers. These novel findings could facilitate design of accurate diagnostic panel for
practical clinical relevance.Methodology - DNA methylation profiles were extracted from TCGA for 418 LUAD and 370 LUSC tissue samples from patients compared with 32 and 42 non-malignant ones respectively. Standard pipeline was conducted to discover significant differentially methylated sites as prim
ary biomarkers. Secondary biomarkers were extracted by incorporating genes associated with comorbidities from meta-analysis of research articles. Concordant candidates were utilized for NSCLC relevant biomarker candidates. Gene ontology annotations were used to calculate gene-pair distance matrix fo
r all candidate biomarkers. Clustering algorithms were utilized to categorize candidate genes into different functional groups using the gene distance matrix. There were 35 CpG loci identified by comparing TCGA training cohort with GEO testing cohort from these functional groups, and 4 gene-based pa
nel was devised after finding highly discriminatory diagnostic panel through combinatorial validation of each functional cluster.Results – To evaluate the gene panel for NSCLC, the methylation levels of SCT(Secritin), FOXD3(Forkhead Box D3), TRIM58(Tripartite Motif Containing 58) and TAC1(Tachikinin
1) were tested. Individually each gene showed significant methylation difference between LUAD and LUSC training cohort. Combined 4-gene panel AUC, sensitivity/specificity were evaluated with 0.9596, 90.43%/100% in LUAD; 0.949, 86.95%/98.21% in LUSC TCGA training cohort; 0.94, 85.92%/97.37 in GEO 66
836; 0.91,89.17%/100% in GEO 83842 smokers; 0.948, 91.67%/100% in GEO83842 non-smokers independent testing cohort. Our study validates SCT, FOXD3, TRIM58 and TAC1 based gene panel has great potential in early recognition of NSCLC undetermined lung nodules. The findings can yield universally accurate
and robust markers facilitating early diagnosis and rapid severity examination.
Acceleration of the Em, MM, and Other Monotone Algorithms for Modern Applications
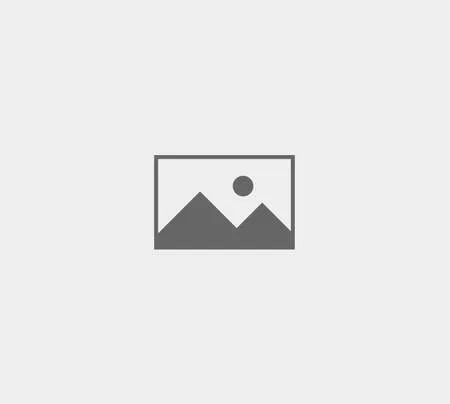
為了解決Oncology 的問題,作者Varadhan, Ravi,Zhou, Hua 這樣論述:
Dr. Varadhan is an Associate Professor of Oncology in the Division of Biostatistics and Bioinformatics at the Sidney Kimmel Comprehensive Cancer Center (SKCCC). He is also a core faculty in the Center on Aging and Health and the Center for Drug Safety and Effectiveness. Dr. Varadhan is a well-known
expert in several areas of biostatistics. He is an expert in the area of patient-centered outcomes research (PCOR), where he focuses on developing statistical methods that inform evidence-based individualized medicine. He has a particular interest in Bayesian methods for exploring heterogeneity of t
reatment effect (HTE). He has developed numerous algorithms and software for solving high-dimensional optimization problems arising in statistical modeling. Hua Zhou teaches and does research in biostatistics at the University of California, Los Angeles (UCLA). She received her Ph.D. in 2008 from th
e Department of Statistics at Stanford University.
以疾病不確定感理論發展整合性心動健康網路照顧模式提升心房顫動病人因應策略之成效探討
為了解決Oncology 的問題,作者謝慧玲 這樣論述:
正文目錄正文目錄『表』目錄 IV『圖』目錄 V『附錄』目錄 VII中文摘要 VIII英文摘要 X第一章 緒論 1 第一節 研究背景、動機及重要性 1 第二節 研究目的 7第二章 文獻查證 8 第一節 心房顫動疾病簡介 8 第二節 疾病不確定感理論 15 第三節 疾病不確定感相關研究 22 第四節 整合性健康網路照顧模式的發展及運用 31第三章 研究架構與假設 36 第一節 研究架構 36 第二節 研究假設 37 第三節 名詞界定 38第四章 研究方法與過程 43 第一節 研究設計 43 第二節 研究對象及場所 45 第三節 研究工具 46
第四節 研究工具之信效度檢定 52 第五節 研究過程 59 第六節 研究倫量 63 第七節 資料處理與統計分析 64第五章 研究結果 66 第一節 心房顫動病人的基本屬性68 第二節 心房顫動病人的症狀困擾、疾病知識、社會支持、疾病不確定感、因應策略及心理困擾之前後測情形 76 第三節 介入「整合性心動健康網路照顧模式」對於心房顫動病人症狀困擾、疾病知識、社會支持、疾病不確定感、因應策略及心理困擾之成效 85第六章 討論 107 第一節 心房顫動病人的基本屬性現況分析 108 第二節 介入「整合性心動健康網路照顧模式」對於改善心房顫動病人症狀困擾之成效 111
第三節 介入「整合性心動健康網路照顧模式」對於改善心房顫動病人疾病知識之成效 113 第四節 介入「整合性心動健康網路照顧模式」對於改善心房顫動病人社會支持之成效 115 第五節 介入「整合性心動健康網路照顧模式」對於改善心房顫動病人疾病不確定感之成效 117 第六節 介入「整合性心動健康網路照顧模式」對於改善心房顫動病人因應策略之成效 119 第七節 介入「整合性心動健康網路照顧模式」對於改善心房顫動病人心理困擾之成效 121 第八節 研究限制 124第七章 結論與建議 125 第一節 結論 125 第二節 建議 127參考文獻 129附錄 141『表』目錄表1. 資料處理
與分析 65表2. 心房顫動病人之人口基本屬性 70表3. 心房顫動病人的疾病特性 74表4. 心房顫動病人症狀困擾、疾病知識、社會支持、疾病不確定感、因應策略及心理困擾之前測與後測結果 83表5. 以 GEE 方法探討整合性心動健康網路照顧模式於心房顫動病人症狀困擾改變之成效 86表6. 以 GEE 方法探討整合性心動健康網路照顧模式於心房顫動病人疾病知識改變之成效 89表7. 以GEE方法探討整合性心動健康網路照顧模式於心房顫動病人社會支持改變之成效 92表8. 以GEE方法探討整合性心動健康網路照顧模式對於心房顫動病人疾病不確定感之改變成效 95表9. 以GEE方法探討整合性心動健康網路
照顧模式對於心房顫動病人因應策略改變之成效 98表10. 以GEE方法探討整合性心動健康網路照顧模式對於心房顫動病人心理困擾改變之成效 103『圖』目錄圖1. 不確定感理論架構 21圖2. 研究架構圖 36圖3. 研究設計 44圖4. 流程圖 67圖5. 兩組在第三版症狀頻率-嚴重程度評估量表之症狀頻率次量表平均分數於前測、後測第一個月、第三個月與第六個月的變化 87圖6. 兩組在心房顫動知識量表平均分數於前測、後測第一個月、第三個月與第六個月的變化 90圖7. 兩組在醫療社會支持量表平均分數於前測、後測第一個月、第三個月與第六個月的變化 93圖8. 兩組在中文版Mishel疾病不確定感量表平
均分數於前測、後測第一個月、第三個月與第六個月的變化 96圖9. 兩組在簡易因應量表之應對因應策略次量表平均分數於前測、後測第一個月、第三個月與第六個月的變化 99圖10. 兩組在簡易因應量表之迴避因應策略次量表平均分數於前測、後測第一個月、第三個月與第六個月的變化 100圖11. 兩組在醫院焦慮憂鬱量表平均分數於前測、後測第一個月、第三個月與第六個月的變化 104圖12. 兩組在醫院焦慮憂鬱量表之焦慮次量表平均分數於前測、後測第一個月、第三個月與第六個月的變化 105圖13. 兩組在醫院焦慮憂鬱量表之憂鬱次量表平均分數於前測、後測第一個月、第三個月與第六個月的變化 106『附錄』目錄附錄一
心房顫動病人基本屬性量表 附錄一附錄二 第三版症狀頻率-嚴重程度評估量表之症狀頻率次量表 附錄二附錄三 心房顫動知識量表 附錄三附錄四 醫療社會支持量表 附錄四附錄五 中文版Mishel疾病不確定感量表 附錄五附錄六 簡易因應量表 附錄六附錄七 醫院憂鬱焦慮量表 附錄七
想知道Oncology更多一定要看下面主題
Oncology的網路口碑排行榜
-
#1.Oncomine Assays for Oncology Research - Thermo Fisher ...
Oncomine assays are multi-biomarker targeted NGS assays designed for cancer research. Analysis of multiple biomarker types—fusions, insertion/deletions ... 於 www.thermofisher.com -
#2.Home - American Society for Radiation Oncology (ASTRO)
American Society for Radiation Oncology · Doctor reading a tablet at a desk. ASTROgram · metal numbers flat on table in clustered group. New on the Blog · Flag, ... 於 www.astro.org -
#3.Oncology - Therapeutic Focus Areas - Our Science | AbbVie
AbbVie in Oncology: Committed to transforming standards of care. We continue to advance our dynamic pipeline to deliver new innovations for cancer patients. 於 www.abbvie.com -
#4.Labcorp Oncology: Diagnostic Testing for Cancer Treatment
Labcorp Oncology provides cancer testing services and genetic testing for cancer predisposition. 於 oncology.labcorp.com -
#5.American Journal of Clinical Oncology - Lippincott
American Journal of Clinical Oncology is a multidisciplinary journal for cancer surgeons, radiation oncologists, medical oncologists, GYN oncologists, ... 於 journals.lww.com -
#6.Advances in Radiation Oncology: Home Page
Founded in 1958, ASTRO's mission is to advance the practice of radiation oncology by promoting excellence in patient care, providing opportunities for ... 於 www.advancesradonc.org -
#7.Journal of Clinical Oncology
Journal of Clinical Oncology (JCO) is a high-impact, peer-reviewed medical journal that publishes significant clinical oncology research along with ... 於 ascopubs.org -
#8.Oncology: Advances & New Cancer Treatments | Pfizer
Pfizer Oncology is transforming the landscape of cancer. We strive to improve the lives of cancer patients with new advancements and treatment developments. 於 www.pfizer.com -
#9.Society of Surgical Oncology
SSO is a dynamic global community of cancer surgeons shaping advancements in the profession to deliver the highest quality surgical care for cancer ... 於 www.surgonc.org -
#10.Oncology – AstraZeneca - Redefining Cancer Care
Our Oncology strategy is built with one goal in mind – to push the boundaries of science to transform the lives of patients living with cancer. Learn more. 於 www.astrazeneca.com -
#11.Oncology - Sanofi
Oncology · Research & Development. Sanofi is investing substantially in the next generation of cancer medicines. · When Cancer Grows Old. This multiyear ... 於 www.sanofi.com -
#12.Definition of oncology - NCI Dictionary of Cancer Terms
NCI's Dictionary of Cancer Terms provides easy-to-understand definitions for words and phrases related to cancer and medicine. 於 www.cancer.gov -
#13.Surgical Oncology - Brigham and Women's Hospital
Learn about BWH's Surgical Oncology for Breast Cancer Surgery; surgical treatment for Cutaneous (Skin Cancer) and Melanoma; Liver, Pancreas and Biliary ... 於 www.brighamandwomens.org -
#14.Medical Oncology | Department of Medicine
The Division of Medical Oncology is a dynamic and rapidly evolving specialty that provides a wide range of opportunities in research, teaching and creative ... 於 deptmedicine.utoronto.ca -
#15.Cancer Treatment & Research | Moffitt
Blood & Bone Marrow Transplant · Cardio-Oncology · Chemotherapy · Clinical Pathways · Clinical Trials · Endoscopic Procedures · Fertility Preservation ... 於 moffitt.org -
#16.Oncology - Roche
Oncology. Our scientists take a rigorous, comprehensive approach to understanding cancer biology and drug discovery. Find out more. 於 www.roche.com -
#17.Medical Oncology - Yale School of Medicine
Welcome to the Section of Medical Oncology in the Department of Internal Medicine at Yale School of Medicine. Our section has a long and rich tradition of. 於 medicine.yale.edu -
#18.Homepage - ESGO - European Society of Gynaecological ...
Europe's Leading Gynaecological Oncology Society. 於 www.esgo.org -
#19.Division of Oncology | Department of Medicine
The UNC Division of Oncology offers specialty expertise in the diagnosis and treatment of a broad spectrum of solid tumors, research activities on cancer ... 於 www.med.unc.edu -
#20.Takeda Oncology
Takeda Oncology - Discovering and developing innovative therapies to deliver potentially transformative treatments. 於 www.takedaoncology.com -
#21.Oncology - Medscape
Oncology : Welcome to Medscape Oncology, where you can peruse the latest medical news, commentary from clinician experts, major conference coverage, ... 於 www.medscape.com -
#22.Radiotherapy and Oncology: Home Page
Radiotherapy & Oncology publishes papers describing original research as well as review articles. It covers areas of interest relating to radiation oncology ... 於 www.thegreenjournal.com -
#23.Radiation Oncology - Los Angeles - Cedars-Sinai
Cedars-Sinai's Radiation Oncology Department designs custom treatment plans in Southern California using the most advanced technologies and therapies. 於 www.cedars-sinai.org -
#24.Oncology Specialty Care Practice Management | McKesson
From smarter comprehensive drug management and oncology clinical management technology to insightful advisors and tools, we're ready to help your practice ... 於 www.mckesson.com -
#25.Department of Medical Oncology - Dana-Farber Cancer Institute
The Department of Medical Oncology provides compassionate clinical care for patients with cancer; conducts transformative basic, translational, and clinical ... 於 www.dana-farber.org -
#26.ESTRO - Home
The European SocieTy for Radiotherapy and Oncology. All rights reserved. ESTRO Avenue Marnix 17, 1000 Brussels . +32 2 775 93 40. 於 www.estro.org -
#27.OncLive - Clinical Oncology News, Cancer Expert Insights
OncLive serves as the connection to oncology, including groundbreaking cancer news and interviews with top oncologists in multimedia formats. 於 www.onclive.com -
#28.Journal of Hematology & Oncology | Home page
First launched in 2008, the Journal of Hematology & Oncology now ranks in the top quartile of journals worldwide in both of these closely intertwined and . 於 jhoonline.biomedcentral.com -
#29.腫瘤學- 維基百科,自由的百科全書
腫瘤學(英語:Oncology)是一種研究腫瘤(尤其是惡性腫瘤)的病因、預防、診斷、治療以及基礎研究的綜合性、獨立性醫學二級學科。 提高腫瘤患者生存率的方法主要包括 ... 於 zh.wikipedia.org -
#30.UCLA Radiation Oncology, Los Angeles, CA.
The UCLA Department of Radiation Oncology pushes back the boundaries that limit ordinary clinical cancer treatment through the application of thoughtful ... 於 www.uclahealth.org -
#31.Texas Oncology – More Breakthroughs. More Victories ...
Texas Oncology is one of the largest cancer treatment and research providers in Texas specializing in hematology, pediatric and radiation oncology and more. 於 www.texasoncology.com -
#32.Oncology - Merck.com
At Merck, we focus on oncology researh to invent a world where cancer isn't just treated, but cured. We follow the science because patients are waiting. 於 www.merck.com -
#33.Oncology - UpToDate
Back Oncology. You receive the entire UpToDate library of specialties with your subscription. Click on a section below to view a detailed list of topics ... 於 www.uptodate.com -
#34.European Urology Oncology: Home Page
Urology, Medical Oncology, Radiation Therapy, Imaging, Pathology and Basic Research working together with the same final aim: to advance research in urological ... 於 euoncology.europeanurology.com -
#35.New York Oncology Hematology: NYOH
Cancer treatment available in New York's Capital District including Saratoga County, providing the latest cancer therapies close to home. 於 newyorkoncology.com -
#36.in Oncology - Frontiers
Frontiers in Oncology- Furthering our insight into carcinogenesis and tumor progression, to bridge the gap between basic research and clinical application. 於 www.frontiersin.org -
#37.National Comprehensive Cancer Network - Home
Recommendations and other materials developed by NCCN Member Institutions or Panel Members to support the oncology community during the ongoing COVID-19 ... 於 www.nccn.org -
#38.Department of Oncology-Pathology | Karolinska Institutet
The Department of Oncology-Pathology conducts basic, translational and clinical research and educational activities related to cancer. 於 ki.se -
#39.Targeted Oncology - Immunotherapy, Biomarkers, and Cancer ...
Targeted Oncology provides news, videos, and reviews on the rapidly evolving world of targeted therapies and immunotherapy for oncologists treating patients ... 於 www.targetedonc.com -
#40.Oncology - Genentech
Focus on Oncology. Genentech has long been a leader in understanding and advancing the fields of cancer biology, cancer immunology and oncology drug ... 於 www.gene.com -
#41.Molecular Oncology - Wiley Online Library
Molecular Oncology is an open access international journal that highlights new discoveries, approaches, and technical developments in basic, clinical, ... 於 febs.onlinelibrary.wiley.com -
#42.Cancer Research and Treatment - IBM and oncology
Oncology is a rapidly evolving discipline with many complex treatment and data challenges. IBM offers medical imaging solutions that can help clinicians ... 於 www.ibm.com -
#43.Oncology Nursing Certification Corporation |
Start now - choose one of the certifications listed below. Certifications for Oncology Nurses. Oncology Certified Nurse (OCN) ... 於 www.oncc.org -
#44.Oncology - MSD
At MSD, we focus on oncology researh to invent a world where cancer isn't just treated, but cured. We follow the science because patients are waiting. 於 www.msd.com -
#45.Radiation Oncology Division - MD Anderson Cancer Center
The mission of MD Anderson's Radiation Oncology Division is to provide quality radiation treatment to cancer patients. Learn more here. 於 www.mdanderson.org -
#46.Journal of Thoracic Oncology: Home Page
As multiple agents are approved for the systemic therapy of lung cancer, controversies have arisen regarding optimal regimens for treatment. 於 www.jto.org -
#47.Annals of Oncology: Home Page
Annals of Oncology, the journal of the European Society for Medical Oncology and the Japanese Society of Medical Oncology, provides rapid and efficient peer- ... 於 www.annalsofoncology.org -
#48.International Journal of Radiation Oncology, Biology, Physics
Founded in 1958, ASTRO's mission is to advance the practice of radiation oncology by promoting excellence in patient care, providing opportunities for ... 於 www.redjournal.org -
#49.Clinical Oncology News
The mission of Clinical Oncology News is to be an independent source of unbiased, accurate and reliable news combined with in-depth expert analysis about ... 於 www.clinicaloncology.com -
#50.The US Oncology Network: Home
Over 20 years of oncology practice management expertise. Patient Care, Practice Health and Physician Satisfaction. 於 usoncology.com -
#51.Hematology Oncology Clinic
Hematology Oncology Clinic. INFORMATION FOR OUR PATIENTS ABOUT COVID-19 (Coronavirus). We understand you may have questions about COVID-19 vaccine availability ... 於 hocbr.com -
#52.Oncologic Conditions Symptoms & Causes | Dignity Health
Oncology is a medical specialty that focuses on diagnosing, treating, and preventing cancer. Cancer occurs when abnormal cells replicate uncontrollably and ... 於 www.dignityhealth.org -
#53.American Society of Clinical Oncology | Choosing Wisely
American Society of Clinical Oncology. Ten Things Physicians and Patients Should Question. Released April 4, 2012 (Items 1 – 5) and October 29, ... 於 www.choosingwisely.org -
#54.Clinical Oncology: Home Page
The Adjudicators, from the Clinical Oncology Editorial Board recommended the following paper for the Frank Ellis Medal Award for 2019. 於 www.clinicaloncologyonline.net -
#55.American Society of Clinical Oncology: ASCO Hub
This site uses tracking technologies through the use of permanent cookies and web beacons/pixel tags. By default, cookies are set to “Allow all cookies.” If you ... 於 www.asco.org -
#56.Japanese Journal of Clinical Oncology | Oxford Academic
A multidisciplinary journal for clinical oncologists which publishes high quality articles on medical oncology, clinical trials, radiology, surgery, ... 於 academic.oup.com -
#57.Oncology - Clinical Care Options
At Clinical Care Options, we offer oncology CME/CE courses for clinicians to stay updated with advanced treatment options and research. Learn more here. 於 www.clinicaloptions.com -
#58.Oncology in Clinical Practice - Via Medica Journals
Oncology in Clinical Practice (OCP) is a peer-reviewed, Open Access journal that features papers on various types of neoplastic disease, including diagnosis ... 於 journals.viamedica.pl -
#59.Children's Oncology Group
CHILDREN'S ONCOLOGY GROUP -- The world's childhood cancer experts. 於 childrensoncologygroup.org -
#60.Oncology - Mayo Clinic Health System
Oncology (Cancer). Our team of experts focuses on the diagnosis, assessment and treatment of various types of cancer, as well as your health needs beyond ... 於 www.mayoclinichealthsystem.org -
#61.Genitourinary (GU) Oncology | Patient Care - Weill Cornell ...
The world-renowned Genitourinary (GU) Oncology Program at Weill Cornell Medicine is dedicated to providing cutting-edge multidisciplinary treatment at every ... 於 weillcornell.org -
#62.Practical Radiation Oncology: Home Page
Founded in 1958, ASTRO's mission is to advance the practice of radiation oncology by promoting excellence in patient care, providing opportunities for ... 於 www.practicalradonc.org -
#63.Oncology Nursing Society | ONS | ons.org
Understanding the underlying mechanisms of cancerrelated symptoms enables oncology nurses to provide the best patient-centered care. Biosignatures are ... 於 www.ons.org -
#64.Novartis Oncology
Discover how Novartis Oncology is driven by one vision: to transform the way patients live with cancer and related diseases. 於 www.novartis.com -
#65.Oncology - Top-Ranked Cancer Care for Children
In our oncology department, Children's National delivers world-renowned pediatric cancer expertise from leukemia to brain tumors. 於 childrensnational.org -
#66.European School of Oncology: ESO
The European School of Oncology was founded by Umberto Veronesi and Laudomia Del Drago in 1982, with the aim of contributing to the reduction of deaths from ... 於 www.eso.net -
#67.Oncology Reports - Spandidos Publications
Oncology Reports is an international journal devoted to fundamental and applied research in Oncology. 於 www.spandidos-publications.com -
#68.European Society for Medical Oncology
ESMO is Europe's leading medical oncology society, providing a professional network for its members and working with national societies across Europe. 於 www.esmo.org -
#69.Types of Oncologists | Cancer.Net
Oncology is the study of cancer. An oncologist is a doctor who treats cancer and provides medical care for a person diagnosed with cancer. 於 www.cancer.net -
#70.Medical Oncology | BIDMC of Boston
BIDMC's Medical Oncology division provides care for various types of cancer. Learn about our services & call 617-667-2100 to schedule a consultation. 於 www.bidmc.org -
#71.DPhil in Oncology | University of Oxford
About the courseThis programme aims to train the next generation of leaders in translational oncology research by applying state-of-the-art techniques to ... 於 www.ox.ac.uk -
#72.Hematology/Oncology Clinics of North America | Journal
Read the latest articles of Hematology/Oncology Clinics of North America at ScienceDirect.com, Elsevier's leading platform of peer-reviewed scholarly ... 於 www.sciencedirect.com -
#73.Oncology Center of Excellence | FDA
The FDA Oncology Center of Excellence leverages the combined skills of regulatory scientists and reviewers with expertise in drugs, biologics, ... 於 www.fda.gov -
#74.Oncology | Cancer Council
Oncology is a sub-specialty of medicine dedicated to the investigation, diagnosis and treatment of people with cancer or suspected cancer. Learn more. 於 www.cancer.org.au -
#75.Orthopedic Oncology - Massachusetts General Hospital
For more than 45 years, the Mass General Orthopaedic Oncology Service has treated children, adolescents and adults with primary bone and soft tissue tumors ( ... 於 www.massgeneral.org -
#76.Current Oncology | An Open Access Journal from MDPI
Recent studies have obtained inadequate data on the association between nutritional status, body composition, clinical parameters and tumor stage in patients ... 於 www.mdpi.com -
#77.Tennessee Oncology
Tennessee Oncology provides access to high-quality cancer care and the expertise of clinical research for all patients, at convenient locations within their ... 於 tnoncology.com -
#78.Hematology and Oncology - 中國醫藥大學附設醫院
We established a center for leukemia research and treatment which was funded by the project of “Center of Excellence in Cancer Research” of Health ... 於 www.cmuh.cmu.edu.tw -
#79.Gastrointestinal Medical Oncology Clinic | UCSF Health
UCSF's Gastrointestinal Medical Oncology Clinic offers the most advanced and effective treatments available for cancerous and benign gastrointestinal ... 於 www.ucsfhealth.org -
#80.JAMA Oncology – Cancer Treatment, Genetics, & Prevention
Explore the latest in cancer science including checkpoint inhibitors, DCIS, breast prostate and lung cancer treatment, aspirin prevention, ... 於 jamanetwork.com -
#81.中華民國癌症醫學會Taiwan Oncology Society
學術活動 · 2021 STSG November Meeting. 110/11/17 18:30 - 110/11/17 20:30 · 衛生福利部癌症研究計畫2021年成果發表暨國際研討會-大數據於癌症預防與治療之應用 · IASGO ... 於 www.taiwanoncologysociety.org.tw -
#82.oncology中文(繁體)翻譯:劍橋詞典
The social worker in a medical oncology department was asked to see the wife of a patient with advanced cancer. 來自Cambridge English Corpus. A brief overview ... 於 dictionary.cambridge.org -
#83.Department of Radiation Oncology - Memorial Sloan Kettering ...
Memorial Sloan Kettering's Department of Radiation Oncology is dedicated to providing superior patient care to our patients while leading in innovation. 於 www.mskcc.org -
#84.Oncology - Nationwide Children's Hospital
Oncology. When your child is diagnosed with cancer, everything changes. ... The Hematology and Oncology Outpatient Clinic is designed to be a link between ... 於 www.nationwidechildrens.org -
#85.Top Oncology Schools in the World - US News Education
See the US News rankings for the world's top universities in Oncology. Compare the academic programs at the world's best universities. 於 www.usnews.com -
#86.MSc Oncology | Chester Medical School Courses
Oncology MSc. This course aims to inform and equip the practitioner with the necessary skills to function in a modern biomedical/clinical environment ... 於 www1.chester.ac.uk -
#87.NRG Oncology
NRG Oncology is making strides to improve the lives of those affected by cancer through practice-changing clinical and translational research. 於 www.nrgoncology.org -
#88.Current issue - The Lancet Oncology
Access articles from The Lancet Oncology, covering topics that advance clinical practice, challenge the status quo, advocate change in health policy, ... 於 www.thelancet.com -
#89.Oncology - Cancer Network
Oncology. home · journals; oncology. The journal ONCOLOGY® provides oncology professionals with practical and timely clinical information necessary to ... 於 www.cancernetwork.com -
#90.Home | JCOG-Japan Clinical Oncology Group
The Japan Clinical Oncology Group (JCOG) is the largest Japanese cooperative group funded primarily by the National Cancer Center Research and Development ... 於 www.jcog.jp -
#91.Journal of Oncology | Hindawi
Journal of Oncology publishes research related to breast cancer, lung cancer, gastrointestinal cancer, skin cancer, head and neck cancer, ... 於 www.hindawi.com -
#92.Japan Society of Clinical Oncology
What's New. archives. September 27, 2021: ESMO Asia Virtual Oncology Week 2021 Registration Now Open! August 17, 2021: KSMO2021 Virtual Meeting Free ... 於 www.jsco.or.jp -
#93.Oncology (Medical) - Department home - Mayo Clinic
Mayo Clinic Oncology — Medical oncologists at Mayo Clinic offer innovative treatments for cancers of the organs, bones, muscles and connective tissues. 於 www.mayoclinic.org -
#94.Department of Oncology - University of Alberta
The Cancer Research Institute of Northern Alberta (CRINA) is a pan-university institute that brings together over 120 researchers from eight faculties. 於 www.ualberta.ca -
#95.Nature Reviews Clinical Oncology
Featuring the best and latest research across the entire spectrum of clinical oncology, ensuring that the work we publish reaches the widest possible ... 於 www.nature.com -
#96.Oncology - Home - Karger Publishers
Oncology. Cover. International Journal of Cancer Research and Treatment. Editor(s): Markman, M. (Philadelphia, PA) 於 www.karger.com -
#97.Annals of Oncology - Journals | Elsevier
Annals of Oncology, the journal of the European Society for Medical Oncology and the Japanese Society of Medical Oncology, provides rapid and efficient ... 於 www.journals.elsevier.com -
#98.Division of Hematology and Oncology - Penn Medicine
The Division of Hematology and Oncology offers comprehensive care for blood disorders and cancer through specialized care and a multidisciplinary approach. 於 www.pennmedicine.org