Selection的問題,我們搜遍了碩博士論文和台灣出版的書籍,推薦Farley, Terri寫的 Phantom Stallion, Wild Horse Island, Volume 35: Galloping Gold 和Todd, Maria K.的 Employer’s Guide to Medical Tourism Benefit Design都 可以從中找到所需的評價。
另外網站110-1Course_Pre-Selection-English.pdf也說明:110-1Course_Pre-Selection-English.pdf. Thumbnails Document Outline Attachments. Find in document… Previous. Next. Highlight all. Match case. Whole words.
這兩本書分別來自 和所出版 。
國立臺北科技大學 電資學院外國學生專班(iEECS) 白敦文所指導 VAIBHAV KUMAR SUNKARIA的 An Integrated Approach For Uncovering Novel DNA Methylation Biomarkers For Non-small Cell Lung Carcinoma (2022),提出Selection關鍵因素是什麼,來自於Lung Cancer、LUAD、LUSC、NSCLC、DNA methylation、Comorbidity Disease、Biomarkers、SCT、FOXD3、TRIM58、TAC1。
而第二篇論文國立中正大學 電機工程研究所 余松年所指導 何亞恩的 一個使用智慧型手機實現深度學習心電圖分類的心臟疾病辨識系統 (2022),提出因為有 智慧型手機即時辨識、心電圖、深度學習、多卷積核模型、注意力機制的重點而找出了 Selection的解答。
最後網站SELECTION PROJECT [1] 線上看 - 巴哈姆特動畫瘋則補充:每年夏天舉辦的,以偶像為目標對於少女們最盛大登龍門「SELECTION PROJECT」,那是以偶像為目標努力著對於所有少女們所憧憬的舞台。
Phantom Stallion, Wild Horse Island, Volume 35: Galloping Gold
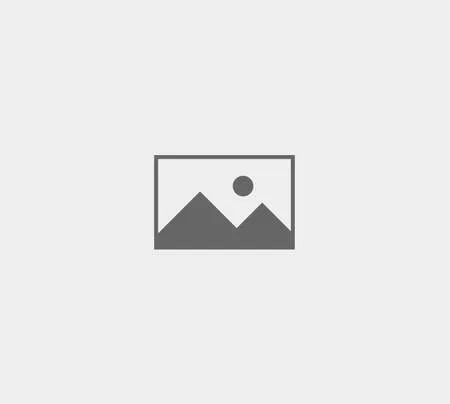
為了解決Selection 的問題,作者Farley, Terri 這樣論述:
Terri Farley is the best-selling author of books about the contemporary and historic West. Her most recent book Wild at Heart: Mustangs and the Young People Fighting to Save Them (Houghton Mifflin Harcourt) is a Junior Library Guild selection; winner of the Sterling North Heritage award for Excel
lence in Children’s Literature; and has been honored by Western Writers of America, National Science Teachers Association, and American Association for the Advancement of Science. She is a recent inductee into the Nevada Writers Hall of Fame. Her Phantom Stallion (HarperCollins) series for young r
eaders has sold over two million copies worldwide.
Selection進入發燒排行的影片
メインの動画はこちら
https://youtu.be/6G0o0UNGcgI
SUSURUのラーメン自販機(SUSURU RAMEN SELECTION)の
設置場所は以下のリンクでご覧ください
https://linktr.ee/susuruselection
今後も全国で展開予定。内容は時期や地域によって異なります。
どれもSUSURUが何十杯も試食して選んだものです。
ラインナップも今後も増える予定!お楽しみに!!
[チャル蔵編集]
■SUSURUのメインチャンネル
https://www.youtube.com/c/SUSURUTV
■SUSURUの1分ラーメン解説
https://www.youtube.com/channel/UCq00sBSrTFpV-0JoWdmMjjw
■SUSURUの個人チャンネル『成田くん』
https://www.youtube.com/channel/UCjUjYIPNI0Mg1_HMquzaemQ
※サブチャンではSUSURU TV.の編集などを担当しているSUSURUチームみんなでワイワイやることが多いです。
■twitter
SUSURU
https://twitter.com/susuru_tv
チャル蔵
https://twitter.com/chal_zou
ノビー
https://twitter.com/nobee32
ファンレターなどの宛先はこちら
〒164-0001
東京都中野区中野3-42-2
SUSURU宛 まで!
※ここにSUSURUは住んでおりません。近隣の方のご迷惑になるので押しかけたりはご遠慮ください。
サブチャンネルも登録よろしくお願いします!
https://www.youtube.com/channel/UCVdjYnmDsyiUcpcryI-k6ZA
#ラーメン自販機 #SUSURU #冷凍ラーメン
An Integrated Approach For Uncovering Novel DNA Methylation Biomarkers For Non-small Cell Lung Carcinoma
為了解決Selection 的問題,作者VAIBHAV KUMAR SUNKARIA 這樣論述:
Introduction - Lung cancer is one of primal and ubiquitous cause of cancer related fatalities in the world. Leading cause of these fatalities is non-small cell lung cancer (NSCLC) with a proportion of 85%. The major subtypes of NSCLC are Lung Adenocarcinoma (LUAD) and Lung Small Cell Carcinoma (LUS
C). Early-stage surgical detection and removal of tumor offers a favorable prognosis and better survival rates. However, a major portion of 75% subjects have stage III/IV at the time of diagnosis and despite advanced major developments in oncology survival rates remain poor. Carcinogens produce wide
spread DNA methylation changes within cells. These changes are characterized by globally hyper or hypo methylated regions around CpG islands, many of these changes occur early in tumorigenesis and are highly prevalent across a tumor type.Structure - This research work took advantage of publicly avai
lable methylation profiling resources and relevant comorbidities for lung cancer patients extracted from meta-analysis of scientific review and journal available at PubMed and CNKI search which were combined systematically to explore effective DNA methylation markers for NSCLC. We also tried to iden
tify common CpG loci between Caucasian, Black and Asian racial groups for identifying ubiquitous candidate genes thoroughly. Statistical analysis and GO ontology were also conducted to explore associated novel biomarkers. These novel findings could facilitate design of accurate diagnostic panel for
practical clinical relevance.Methodology - DNA methylation profiles were extracted from TCGA for 418 LUAD and 370 LUSC tissue samples from patients compared with 32 and 42 non-malignant ones respectively. Standard pipeline was conducted to discover significant differentially methylated sites as prim
ary biomarkers. Secondary biomarkers were extracted by incorporating genes associated with comorbidities from meta-analysis of research articles. Concordant candidates were utilized for NSCLC relevant biomarker candidates. Gene ontology annotations were used to calculate gene-pair distance matrix fo
r all candidate biomarkers. Clustering algorithms were utilized to categorize candidate genes into different functional groups using the gene distance matrix. There were 35 CpG loci identified by comparing TCGA training cohort with GEO testing cohort from these functional groups, and 4 gene-based pa
nel was devised after finding highly discriminatory diagnostic panel through combinatorial validation of each functional cluster.Results – To evaluate the gene panel for NSCLC, the methylation levels of SCT(Secritin), FOXD3(Forkhead Box D3), TRIM58(Tripartite Motif Containing 58) and TAC1(Tachikinin
1) were tested. Individually each gene showed significant methylation difference between LUAD and LUSC training cohort. Combined 4-gene panel AUC, sensitivity/specificity were evaluated with 0.9596, 90.43%/100% in LUAD; 0.949, 86.95%/98.21% in LUSC TCGA training cohort; 0.94, 85.92%/97.37 in GEO 66
836; 0.91,89.17%/100% in GEO 83842 smokers; 0.948, 91.67%/100% in GEO83842 non-smokers independent testing cohort. Our study validates SCT, FOXD3, TRIM58 and TAC1 based gene panel has great potential in early recognition of NSCLC undetermined lung nodules. The findings can yield universally accurate
and robust markers facilitating early diagnosis and rapid severity examination.
Employer’s Guide to Medical Tourism Benefit Design
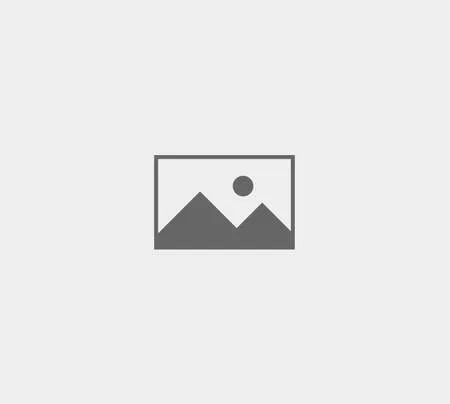
為了解決Selection 的問題,作者Todd, Maria K. 這樣論述:
Health travel, domestic and international, for the group health benefit sector is an established cost containment option that was for years, used primarily by reinsurers and case management firms and limited to rare, high-cost, tertiary care. Through the use of cost-saving benefit design incentives,
employers are testing the receptiveness of plan participants and encouraging plan members to consider a narrow network of high-performance healthcare providers in targeted locations that may be located further from home. In addition to foreign medical tourism, this has given rise to another emergin
g market - domestic medical tourism. Unlike foreign medical tourism, patients don't leave the country. Instead they travel to another city with the U.S. to have procedures for upt to 75% less than they would pay if they were treated closer to home. Large employers such as Wal-Mart, Lowe's and Pepsi
Co are offering employees and dependents heart, spine and transplant surgeries at large medical facilities such as John Hopkins and the Cleveland Clinic, regardless of where they are located in the U.S. This book addresses how to design and launch a health travel benefit pilot program, plan funding
options, quality, safety and logistic considerations, provider selection criteria, and bundled case rate contracting in the USA and abroad. The author also includes many worksheets, checklists and forms to use when designing a health travel benefit program.
一個使用智慧型手機實現深度學習心電圖分類的心臟疾病辨識系統
為了解決Selection 的問題,作者何亞恩 這樣論述:
目錄誌謝 i摘要 iiAbstract iii目錄 v圖目錄 viii表目錄 xi第一章 緒論 11.1研究動機 11.2研究目的 21.3研究架構 2第二章 研究背景 32.1心電圖與疾病介紹 32.1.1心臟導程 32.1.2心臟疾病介紹 52.2Android系統 102.2.1 Android的基礎 102.2.2 Android系統框架 102.3相關文獻探討 11第三章 研究方法 173.1資料庫介紹 173.2訊號前處理 193.2.1小波濾波 193.2.2訊號正規化 213.3一維訊號轉二維影像 213.3.1手機螢幕上
繪製圖形 213.3.2影像儲存於智慧型手機 233.3.3資料擴增Data Augmentation 243.4深度學習架構 253.4.1多卷積核架構 253.4.2注意力模型 283.4.2.1通道注意力模組Channel attention 293.4.2.2空間注意力模組Spatial attention 303.4.2.3激活函數Activation function 303.5損失函數Loss function 313.6交叉驗證Cross validation 323.7優化訓練模型 333.8移動端應用 343.9硬體設備、軟體環境與開發環境 36
3.9.1硬體設備 363.9.2軟體環境與開發環境 37第四章 研究結果與討論 3834.1評估指標 384.2訓練參數設定 404.3實驗結果 414.3.1深度學習模型之辨識結果 414.3.1.1比較資料擴增前後之分類結果 414.3.1.2不同模型架構之分類結果 424.3.2智慧型手機應用結果 464.4相關文獻比較 48第五章 結論與未來展望 525.1結論 525.2未來展望 53參考文獻 54
想知道Selection更多一定要看下面主題
Selection的網路口碑排行榜
-
#1.Grundfos Product Center | Sizing and selection of pumps and ...
Grundfos Product Center is your complete solution for sizing, selection and configuration tools, quick dimensioning, pump replacement, liquid guide, ... 於 product-selection.grundfos.com -
#2._Application.Selection Property (Microsoft.Office.Interop.Word ...
Returns the Selection object that represents a selected range or the insertion point. 於 docs.microsoft.com -
#3.110-1Course_Pre-Selection-English.pdf
110-1Course_Pre-Selection-English.pdf. Thumbnails Document Outline Attachments. Find in document… Previous. Next. Highlight all. Match case. Whole words. 於 dba.asia.edu.tw -
#4.SELECTION PROJECT [1] 線上看 - 巴哈姆特動畫瘋
每年夏天舉辦的,以偶像為目標對於少女們最盛大登龍門「SELECTION PROJECT」,那是以偶像為目標努力著對於所有少女們所憧憬的舞台。 於 ani.gamer.com.tw -
#5.DR.Selection官方旗艦店, 線上商店 - 蝦皮購物
DR.Selection官方旗艦店▻醫美級濃度專家提供您簡單保養概念◅ DR.Selection百分之百於日本バイオテック皮膚研究中心所研發,結合了日本專業實驗室以及台灣醫學美容生 ... 於 shopee.tw -
#6.CCU Course Selection System
NOT available for course selection. PC中文版 行動版(beta version) English version. Student ID: Password: Fall semester of 2021. CCU Course Selection System 於 kiki.ccu.edu.tw -
#7.synonyms for selection - Thesaurus.com
Find 15 ways to say SELECTION, along with antonyms, related words, and example sentences at Thesaurus.com, the world's most trusted free thesaurus. 於 www.thesaurus.com -
#8.selection - 讀音_用法_例句 - 海词词典
That shop has a fine selection of cakes. 那家商店有各種精美的糕點供我們選擇。 That store has a good selection of furniture. 那家店有很多傢具可供挑選。 於 dict.cn -
#9.Reproduction & Selection 複製與選擇(2) - iT 邦幫忙
昨天跟大家提到了隨機選擇(Random Selection Schema)、分裂選擇(Disrupt Selection Schema)、機率選擇(Proportionate Selection Schema)和菁英選擇這幾種那今天 ... 於 ithelp.ithome.com.tw -
#10.selection - Dreye權威釋義
Biology the evolutionary process which determines which types of organism thrive; natural selection. 以上來源於:《簡明牛津英語詞典》 ... 於 yun.dreye.com -
#11.selection - 遴選 - 國家教育研究院雙語詞彙
selection. 以selection 進行詞彙精確檢索結果. 出處/學術領域, 英文詞彙, 中文 ... 於 terms.naer.edu.tw -
#12.Element selection for crystalline inorganic solid discovery ...
The selection of the elements to combine delimits the possible outcomes of synthetic chemistry because it determines the range of ... 於 www.nature.com -
#13.2021年Natural Selection巡迴賽蓄勢待發!Travis Rice及選手 ...
一眾來自世界各地的精英滑雪選手將於2021年2月雲集在美國懷俄明州Jackson Hole Mountain度假村所舉行的Natural Selection巡迴賽! 於 www.redbull.com -
#14.Course Selection Online 國立臺南大學選課系統網站- National
大學部學生選課及課程相關問題,請洽教務教學業務組分機221~225. 2. 碩博生選課及課程相關問題,請洽教務處研究生教務組分機231-232. 3. 通識課程及選課問題,請洽通識 ... 於 academics.nutn.edu.tw -
#15.selection | meaning of selection in Longman Dictionary of ...
selection se‧lec‧tion /səˈlekʃən/ noun · 1[uncountable] the careful choice of a particular person or thing from a groupThe Health Authority is not involved in ... 於 www.ldoceonline.com -
#16.雜誌>日文雜誌索引>Chara Selection - 博客來
博客來 · 雜誌 · 日文雜誌索引; Chara Selection. 顯示模式顯示模式圖文模式. 排序依. 上市日期(新→舊), 上市日期(舊→新) · 暢銷度 · 價格(高→低) · 價格(低→高) ... 於 www.books.com.tw -
#17.Public Enterprises Selection Board: Home
Selection for the post of Director (Technical), ECIL held on 12th October 2021. Highlight Img. More... 於 pesb.gov.in -
#18.selection - CSS(层叠样式表)
selection CSS伪元素应用于文档中被用户高亮的部分(比如使用鼠标或其他选择设备选中的部分)。 於 developer.mozilla.org -
#19.WONDERFUL HOBBY SELECTION | GOOD SMILE COMPANY
WONDERFUL HOBBY SELECTION ワンホビセレクション(WONDERFUL HOBBY SELECTION)は、グッドスマイルカンパニーがお送りする、フィギュア. 於 www.goodsmile.info -
#20.登錄|線上註冊| Monde Selection品質獎項
Submitting your product to Monde Selection is very easy. Fill out the form corresponding to your product category and follow the instructions to send us ... 於 www.monde-selection.com -
#21.Genetics, Selection, Evolution | 標準期刊縮寫(ISO4)
Genetics, Selection, Evolution 的ISO4標準期刊縮寫為Genet. Sel. Evol.。簡單的說,當您需要引用期刊Genetics, Selection, Evolution時,符合ISO4標準規定的國際通用 ... 於 academic-accelerator.com -
#22.Postdoctoral Selection Guidelines - APPIC
APPIC Postdoctoral Selection Guidelines: APPIC strongly encourages Postdoctoral Programs and Applicants to adhere to the following Guidelines for the 2021-2022 ... 於 www.appic.org -
#23.SELECTION PROJECT - 维基百科,自由的百科全书
《SELECTION PROJECT》,日文簡稱「セレプロ」(SelePro),是由KADOKAWA和動畫工房合作推出的日本跨媒體企劃,電視動畫於2021年10月1日首播。 於 zh.wikipedia.org -
#24.選擇排序(Selection Sort) - 寫點科普Kopuchat
選擇排序(Selection Sort). 演算法筆記 • 程式教學. Written by: Lynn. 2017-06-20. 選擇排序的原理是每次都在剩下的資料中找出最小的資料,將該資料丟到當前的正確 ... 於 kopu.chat -
#25.酒如坊Spirits Shop' Selection
酒如坊Spirits Shop' Selection. 於 www.spirits.com.tw -
#26.Addressing Selection Criteria - University of Melbourne
The key selection criteria are found in the Position Description. It clearly documents the qualifications, skills, attributes and knowledge a person must ... 於 about.unimelb.edu.au -
#27.selection是什么意思? selection翻译(中文英文) - 抓鸟
selection 的解释是:选择, 挑选, 选集, 精选品… 同时,该页为英语学习者提供:selection的中文翻译、英英详解、单词音标、在线发音、例句等。 於 dict.zhuaniao.com -
#28.SELECTION TOOLS | Amtrol
IMPORTANT: The AMTROL Selection Tool should be used as a general guide to sizing AMTROL products. Sizing calculations are dependent on user input and should ... 於 www.amtrol.com -
#29.Natural Selection_天擇- Mutation - PhET 互動式模擬教學 - PhET
我們正在努力改進網站的可用性。若您支持我們,請更新您的設定檔。 暫時跳過. 更新設定檔 · 關於PhET 我們的團隊 贊助單位 Partnerships · 離線版 Help Center 聯繫. 於 phet.colorado.edu -
#30.Lucas Selection 盧卡斯選物官方網站
Lucas Selection嚴選世界各地的生活良品,呈獻日本進口、日本設計、香港設計潮牌衣服服飾。同時推薦13Fishing釣具,介紹釣竿、推薦捲線器、路亞等高品質用品。 於 www.lucia-lucas.com -
#31.寧淨選品•生活NJS Ninjin Selection - Instagram
1186 Followers, 19 Following, 107 Posts - See Instagram photos and videos from 寧淨選品•生活NJS Ninjin Selection (@ninjin.selection) 於 www.instagram.com -
#32.金博家jinbo-selection.com.tw
榮獲法國藍帶美食協會評選台灣第一,媽咪速玲「蔥蔥回魂麵」吃過才知名不虛傳!讓人不吃辣卻一口接一口;前所未有的Q彈麵條口感,加上大把蔥花誘人香味,秘制醬料讓你 ... 於 www.jinbo-selection.com.tw -
#33.DR.Selection賽萊斯,品牌總覽(AZ),醫美保養品牌,彩妝保養
DR.Selection賽萊斯,品牌總覽(A-Z),醫美保養品牌,彩妝保養,BIODERMA,Numis med德國樂美思,台糖詩丹雅蘭各式規格種類,與Bioderma,德國樂美思Numis med,Suntory 三得利 ... 於 www.momoshop.com.tw -
#34.How we select - Gates Cambridge Scholarship
Gates Cambridge uses a three-stage selection process to ensure Scholars meet all of our criteria to the highest degree. 於 www.gatescambridge.org -
#35.Careers with the European Union | by the European ...
EPSO is currently undergoing a thorough transformation process for staff selection to better respond to the evolving needs of the EU institutions and the ... 於 epso.europa.eu -
#36.Course Selection System - 國立臺灣科技大學教務處
Course Selection System. Course Selection System · Orientation for Course Selection System · Course Query System & Course Selection System Operating Manual. 於 www.academic.ntust.edu.tw -
#37.selection中文, selection是什麼意思:選擇… - 查查綫上辭典
selections from great poets 名家詩選。 mass selection 混合選種。 artificial [reproductive, sexual] selection 人為[生殖,雌雄]淘汰。 natural selection 自然 ... 於 tw.ichacha.net -
#38.selection - 英文詞性必勝秘笈-名詞
selection. [si'lekʃən]. n.選擇, 挑選, 挑選出來的人或事. 例句與用法:. And now for your further delectation, we present a selection of popular melodies. 於 en995.com -
#39.JKSSB
Notification regarding Final Selection List of District Cadre posts of Class IV Item No. 36,37,38,39,40,41.42,43,44 & 45 Advertised vide Notification No. 於 www.jkssb.nic.in -
#40.SPECIAL SELECTION - midori
SPECIAL SELECTION. 首頁SPECIAL SELECTION. 所有商品 · 熱門商品 · 新商品 · 特價商品. 5商品. New · 選擇規格 加入收藏清單 · ✶獨家販售✶Allforhome X Midori聯名 ... 於 midorikr.com -
#41.selection中文(繁體)翻譯:劍橋詞典
selection 的例句. selection. Unfortunately, the texts of nine of the selections are not printed in the booklet, including all of the pieces ... 於 dictionary.cambridge.org -
#42.CSS沒有極限- CSS藏頭詩Selection | 卡斯伯Blog - 前端
上面這一段讓底色可以變黑,文字變成白色,但是我們可以注意到陰影在反白後,會影響閱讀。 div &::-moz-selection background: black color: white &:: ... 於 wcc723.github.io -
#43.Schedule of Course Selection-CJCU Registration and ...
Stage, Duration. 110-1 Course inquiry, 2021/6/7(08:00). Preliminary selection, 2021/6/15(08:00)~2021/6/22(17:00). Add/Drop Course Selection, Stage 1 ... 於 dweb.cjcu.edu.tw -
#44.國立臺灣大學管理學院國際事務室Course Selection
Usually, the online course selection session commmerces a month prior to the beginning of the semester. To begin with the course selection, students are ... 於 management.ntu.edu.tw -
#45.BS視角.BSelection.設計師視角.品牌推薦
【Brian Selection.BS視角】追隨設計師視角,獵豔無國界的華美。品味設計師為您凝聚的國內外優質品牌。 於 www.bselection.com -
#46.Definition of selection - Merriam-Webster
Definition of selection · 1 : the act or process of selecting : the state of being selected · 2 : one that is selected : choice also : a ... 於 www.merriam-webster.com -
#47.7-series-product-selection-guide.pdf - Xilinx
Notes: 1. Supports PCI Express Base 2.1 specification at Gen1 and Gen2 data rates. 2. Represents the maximum number of transceivers available. 於 www.xilinx.com -
#48.Museum Selection | Collection of Home Accessories ...
Buy furniture, home furnishings and home accessories from Museum Selection. Our range of period mahogany furniture, traditional home furnishings & home ... 於 www.museumselection.co.uk -
#49.學生網路選課系統
Course selection system will screen by the "Mode" and "Size of Limit" of each course. Drop Courses Before Course Add/Drop, 2021/9/22 (Wednesday) 12:30 ... 於 sys.ndhu.edu.tw -
#50.Dynabeads Products Selection Guide - TW
Please choose a category. Cell isolation; T Cell activation and expansion; Exosome isolation and detection; Virus enrichment; Protein isolation (His tag/IP) ... 於 www.thermofisher.com -
#51.selection 的中文翻譯| 英漢字典
selection 選擇,選舉,選拔,挑選;淘汰. 於 cdict.net -
#52.什麼是國際品質標章Monde Selection ?食品界的奧斯卡獎
在電影業界最高殊榮莫過於奧斯卡金像獎,而在質量屆則不外乎是國際品質標章特級金獎Monde Selection Grand Gold Quality Award,這個最高的榮耀在評選 ... 於 www.daikenshop.com -
#53.Modeling and variable selection in epidemiologic analysis
Special attention is given to the task of model selection, which involves selection of the model form, selection of the variables to enter the model, ... 於 pubmed.ncbi.nlm.nih.gov -
#54.How to save and reuse a selection | Adobe Photoshop 教學課程
Save a selection. Make a selection using any of the selection tools or methods. To save this selection, choose Select > Save Selection. 於 helpx.adobe.com -
#55.selection的中文翻譯和情景例句 - 留声词典
N-UNCOUNT選拔;挑選;選擇. Selection is the act of selecting one or more people or things from a group. ...Darwin's principles of natural selection. 於 dictionary.liushengyingyu.com -
#56.K.SELECTION品牌訂製
K.SELECTION品牌標系列. K.SELECTION品牌標系列. 抱歉,這個商品類別沒有相關商品. 建議您,選擇其他分類或者使用關鍵字搜尋 ... 於 www.kselection.com.tw -
#57.DR.Selection 賽萊斯|品牌總覽 - 東森購物
推薦各種DR Selection 賽萊斯品牌總覽例如DR Selection賽萊斯保濕抗老雙面膜10片組玻尿酸天絲面膜5片角鯊黑面膜5片DR Selection賽萊斯玻尿酸舒緩保濕天絲棉面膜1. 於 www.etmall.com.tw -
#58.Selection guide for LIF - 開放式光學尺 - 海德漢
Model, Basline error, Substrate and mounting, Interpolation error, Measuring length. Accuracy grade, Intervall. LIF 400, ± 1 μm 於 www.heidenhain.tw -
#59.DR.Selection 修復- POYA Buy 寶雅線上買
POYA Buy 寶雅線上買為您打造精采生活!提供DR.Selection 修復相關商品便利的線上購物體驗,讓您一次購足所需的DR.Selection 修復等生活用品。 於 www.poyabuy.com.tw -
#60.Micro Chip Fuse selection - Industrial Devices & Solutions
Micro Chip Fuse selection tool. In the past, selecting a Micro Chip Fuse used to require a complicated procedure involving calculations based on service ... 於 industrial.panasonic.com -
#61.HCP Pumps Selection System 河見泵浦選型系統
Qucikly online search and pump selection platform assist you to instantly get your model information. © 2019 HCP PUMPS SELECTION SYSTEM. 於 e-selection.hcppump.com.tw -
#62.AN-29 - TOPSwitch-GX Flyback Quick Selection Curves
AN-29 - TOPSwitch-GX Flyback Quick Selection Curves. TOPSwitch-GX Flyback Quick Selection Curves. 檢視PDF 下載PDF. 產品. TOPSwitch-GX. 於 www.power.com -
#63.臺灣菸酒股份有限公司Monde Selection獲獎紀錄 - 政府資料 ...
Loading... 哈囉!我是客服小幫手,請問你今天想詢問哪一方面的問題呢? 資料集 臺灣菸酒股份有限公司Monde Selection獲獎紀錄. 於 data.gov.tw -
#64.Guidelines for selection of SSSIs | JNCC
Introduction. The Guidelines for the Selection of Biological Sites of Special Scientific Interest (SSSIs) provide a consistent rationale for ... 於 jncc.gov.uk -
#65.product> solutions > pac > xpac-atom > selection guide
Standard XP-9000-WES7 (Windows Embedded Standard 7 Inside) The controller supports following software development tools: 1. DLLs of I/O modules for VS. 於 www.icpdas.com -
#66.Selection shop | 精品嚴選 - HUGHUG
Selection shop | 精品嚴選|. 排列方式. 特色, 按字母順序, A-Z, 按字母順序, Z-A, 價錢, 從低到高, 價錢, 從高到低, 日期, 從新到舊, 日期, 從舊到新 ... 於 www.hugxhug.com.tw -
#67.Option, selection, alternative and choice 四个表示“选择”的单词
虽然selection 在表示“挑选”的意思时和其它三个词语不同,但是它在表示“可选择的事物”的时候,和choice 却有相同之处。咱们先来听两个例句。 Examples The ... 於 www.bbc.co.uk -
#68.國立故宮博物院>典藏精選
繪畫 · 書法 · 圖書 · 文獻 · 陶瓷 · 銅器 · 玉器 · 珍玩 · 新石器時代大汶口文化. 西元前4300-2500. 共3 件. 紅山文化晚期. 西元前3500-3000. 共7 件 ... 於 theme.npm.edu.tw -
#69.1.13. Feature selection — scikit-learn 1.0.1 documentation
feature_selection module can be used for feature selection/dimensionality reduction on sample sets, either to improve estimators' accuracy scores or to boost ... 於 scikit-learn.org -
#70.selection / 9月秋裝直播 - 首頁New in / 秋裝新品Korea / 即時 ...
selection / 9月秋裝直播. 加入購物車. 優雅層次紗短洋裝. NT$2,480 · 加入購物車. 寬領不修邊配色文字長版棉質T. NT$1,580 · 加入購物車. 惡霸犬配色圖案棉質上衣. 於 www.hiloca.co -
#71.The Selection (The Selection, 1): Cass, Kiera: Books - Amazon ...
For thirty-five girls, the Selection is the chance of a lifetime. The opportunity to escape a rigid caste system, live in a palace, and compete for the ... 於 www.amazon.com -
#72.Talent Selection Specialist|台灣積體電路製造股份有限公司 ...
... best-fit candidates through tsmc's selection process, prepare employment proposals, close offers and facilitate the onboarding process. 於 www.104.com.tw -
#73.(DR.Selection 賽萊斯)凌琍國際股份有限公司 - 1111人力銀行
(DR.Selection 賽萊斯)凌琍國際股份有限公司|日系醫美級原液提供您健康保養概念DR.Selection與日本バイオテック皮膚研究中心共同研發,結合了日本專業實驗室以及台灣 ... 於 www.1111.com.tw -
#74.2021 Product Selection Guide - IEI Integration Corp.
2021 IEI product selection guide, including embedded system, panel PC, network appliance, storage server, etc. 於 www.ieiworld.com -
#75.selection | biology | Britannica
selection, in biology, the preferential survival and reproduction or preferential elimination of individuals with certain genotypes (genetic compositions), ... 於 www.britannica.com -
#76.SELECTION PROJECT第1集|免費線上看|動漫 ... - LINE TV
《SELECTION PROJECT|第1集》於每年夏天舉辦,對目標成為偶像的少女們來說最大的成名途徑 ... 於 www.linetv.tw -
#77.SET selection requirements, process and application | RACS
Selection into SET is a competitive process which occurs annually. Applications are scored and applicants with the highest scores are offered places in the ... 於 www.surgeons.org -
#78.Home | Staff Selection Commission | GoI
This is Official Website of Staff Selection Commission. 於 ssc.nic.in -
#79.國立政治大學校務行政系統選課系統NCCU Administration ...
經營管理碩士學程(EMBA)選課系統由此進:. (限Chrome瀏覽器) http://www.emba.sys.nccu.edu.tw/. ‧, 校際選課申請系統(本校生) [操作手冊]. 於 selectcourse.nccu.edu.tw -
#80.Diversity Visa Program - Selection of Applicants - Travel.gov
Each year, the Department of State conducts a random selection of Diversity Immigrant Visa (DV) applicants, based on allocations of available visas in each ... 於 travel.state.gov -
#81.selection - Yahoo奇摩字典搜尋結果
選擇;選拔[U];被挑選出的人(或物);精選品;選手[C]. Dr.eye 譯典通 · selection · 查看更多. IPA[sɪˈlekʃn]. 美式. 英式. n. 挑選;被選中者. 牛津中文字典 ... 於 tw.dictionary.yahoo.com -
#82.Selection Indicators - Millennium Challenge Corporation
Indicator Category Source Access to Credit Indicator Economic Freedom International Monetary Fund; World Bank Business Start‑Up Indicator Economic Freedom World Bank Child Health Indicator Investing in People Columbia/Yale 於 www.mcc.gov -
#83.Maxon Selection Program
Maxon Selection Program. Do you really want to exit this site? Confirm with OK to go to the classic maxon website. It has not been optimized for smartphones ... 於 www.maxongroup.com -
#84.selection (【名詞】可供挑選的東西)意思、用法及發音 - Engoo
The selection process is very competitive, says Rini Paiva, who oversees the selection committee, "because there are a lot of terrific inventors out there ... 於 engoo.com.tw -
#85.主題精選|Selection - ASICS 台灣亞瑟士官方購物網站
主題精選|Selection. 篩選. 請選擇. 排序. 共0 件商品. 品牌故事 · 購物說明 · 客服留言. © 2021 by 台灣亞瑟士股份有限公司. 本網站中使用cookie,欲查詢有關本網站 ... 於 www.asics.com.tw -
#86.Selection Sort - GeeksforGeeks
The selection sort algorithm sorts an array by repeatedly finding the minimum element (considering ascending order) from unsorted part and ... 於 www.geeksforgeeks.org -
#88.永心鳳茶Yonshin Tea & Cake Selection Bar - Home | Facebook
永心鳳茶Yonshin Tea & Cake Selection Bar · October 2 at 3:30 PM · . l 敲碗很久的!永心茶包禮盒重磅登場l . 從試茶、設計、包材挑選到成品,我們的茶包禮盒終於在 ... 於 www.facebook.com -
#89.DR.Selection 賽萊斯
效果。濃度最短時間呵護您每一寸肌膚. 於 dr-s.com.tw -
#90.有沒有逆選擇(Adverse Selection)及道德風險(Moral ...
當市場參與者間存在資訊不對稱,將會產生逆選擇(Adverse Selection)及道德風險(Moral Hazard)之情形。 「逆選擇」是在「事前」(契約簽訂或交易完成前)因交易雙方 ... 於 master.get.com.tw -
#91.第30 屆台灣精品選拔
台灣精品獎是臺灣產業界的奧斯卡獎,獲獎產品都是通過經濟部選聘各類領域專家, 從研發、設計、品質、行銷全方位嚴選出來的產業菁英。 獲獎者除能使用「台灣精品標誌」 ... 於 www.taiwanexcellence.org -
#92.Official Website of Delhi Subordinate Services Selection ...
Welcome to Delhi Subordinate Services Selection Board. LINK FOR ONLINE APPLICATION REGISTRATION SYSTEM (OARS) · Link for Online Exam Mock Test. 於 dsssb.delhi.gov.in -
#93.On-line Course Selection - National Formosa University - 國立 ...
And now you can log in the course selection system at http://ecourse.sys.nfu.edu.tw/. The account name is “your student ID number”, and password is the same ... 於 oia.nfu.edu.tw