sad pronunciation的問題,透過圖書和論文來找解法和答案更準確安心。 我們找到下列包括賽程、直播線上看和比分戰績懶人包
sad pronunciation的問題,我們搜遍了碩博士論文和台灣出版的書籍,推薦Adamek, Piotr寫的 A Good Son Is Sad If He Hears the Name of His Father: The Tabooing of Names in China As a Way of Implementing Social Values 和Walters, Richard (CRT)的 Standard Vocal Literature: Mezzo-Soprano都 可以從中找到所需的評價。
另外網站Google Traductor也說明:El servicio gratuito de Google traduce al instante palabras, frases y páginas web del inglés a más de cien idiomas.
這兩本書分別來自 和所出版 。
國立中央大學 資訊工程學系 王家慶所指導 秦餘皞的 音樂及語音情緒模型建立之研究 (2016),提出sad pronunciation關鍵因素是什麼,來自於情緒辨識、音樂、語音、機器學習。
而第二篇論文國立臺灣大學 應用力學研究所 劉佩玲所指導 游志源的 應用貝氏網路及適應性調適方法於語音情緒辨識之研究 (2010),提出因為有 語音情緒辨識、特徵參數、正規化、多層貝氏網路、多層共變異數貝氏網路、適應性的重點而找出了 sad pronunciation的解答。
最後網站"I'm sad. (pronunciation)"用韓語要怎麼說? | HiNative則補充:I'm sad. (pronunciation) 用韓語要怎麼說? 查看翻譯 · 난슬프다 · 난슬퍼..
A Good Son Is Sad If He Hears the Name of His Father: The Tabooing of Names in China As a Way of Implementing Social Values
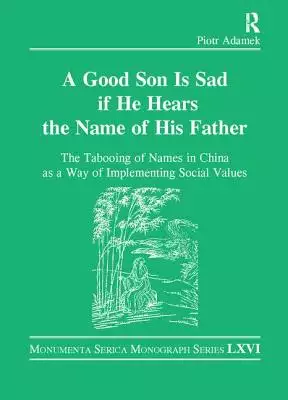
為了解決sad pronunciation 的問題,作者Adamek, Piotr 這樣論述:
When in 1775 the scholar Wang Xihou 王錫侯 compiled a dictionary called Ziguan 字貫, he wrote, for illustrative purposes, the personal names of Confucius and the three emperors Kangxi, Yongzheng and Qianlong in the introduction. In oversight, he recorded their complete names. This accidental writing o
f a few names was condemned by Emperor Qianlong as an unprecedented crime, rebellion and high treason. Wang Xihou was executed, his property confiscated and his books were burnt. His family was arrested and his sons and grandsons were killed or sent as slaves to Heilongjiang. It is surprising what a
n enormous impact the tabooing of names (bihui 避諱) had on Chinese culture. The names of sovereigns, ancestors, officials, teachers, and even friends were all considered taboo, in other words it was prohibited to pronounce them or to record them in writing. In numerous cases characters identical or s
imilar in writing or pronunciation were often avoided as well. The tabooing of names was observed in the family and on the street, in the office and in the emperor's palace. The practice of bihui had serious consequences for the daily lives of the Chinese and for Chinese historiography. People even
avoided certain places and things, and refused to accept offices. They were punished and sometimes even killed in connection with the tabooing of names. The bihui custom existed as an important element of Chinese culture and was perceived as significant by Chinese and foreigners alike. It was crucia
l for implementing social values and demonstrating the political hierarchy. The present work A Good Son Is Sad if He Hears the Name of His Father is a systematic study of Chinese name-tabooing customs, which until now have been relatively little explored in Western-language Sinological studies. It a
ttempts to provide a long-term perspective on the changing dynamics of tabooing and elucidates various aspects related to the fascinating topic of tabooing of names.
sad pronunciation進入發燒排行的影片
😀 Join Hanzi MasterClass Waitlist - Get more updates and info.
about learning Chinese characters.
https://winning-thinker-7590.ck.page/84aef16ed5
FREE Worksheet Download HERE😀
https://smartmandarin.lpages.co/talk-about-emotions-in-chinese
😀 3 Steps To Master Chinese Pronunciation - Free Training
https://katrinalee.easywebinar.live/event-registration-15
😀 Learn 30 Common Chinese Radicals instatnly! FREE Guide!
https://smartmandarin.lpages.co/how-to-learn-hanzi-podcast-worksheet
Let's Get Connected! 😀
SMART Mandarin Faceboook Group
https://www.facebook.com/groups/319018825960625/
Listen to SMART Mandarin on Spotify
Follow SMART Mandarin on Instagram
smart_mandarin_katrina_lee
--------------------------------------------------------------------------
Suggested Video Lessons 😀
How To Cook Egg Fried Rice In Chinese
https://www.youtube.com/watch?v=L6HQb5ncMCA&t=731s
Chinese Daily Use Sentences for Beginners
https://www.youtube.com/watch?v=gcJip1otw98&t=152s
How to Ask Favor in Mandarin
https://www.youtube.com/watch?v=Nya1RBfG5Wc
Don’t’ Understand Mandarin?! Speak Too FAST!!
https://www.youtube.com/watch?v=N3oTjKcHp0Y&t=659s
How to Learn Chinese Characters
https://www.youtube.com/watch?v=k4B9JKrDdtA&t=1457s
Useful Chinese Slangs
https://www.youtube.com/watch?v=ln0U0v7ee38&t=364s
Airport Mandarin
https://www.youtube.com/watch?v=own9Kxc8E18
Useful Chinese Phrases
https://www.youtube.com/watch?v=ybsVmasSfbo
How to Remember Chinese Tones
https://www.youtube.com/watch?v=SQLPOXe8C6s
Super Easy Mandarin for Beginners
https://www.youtube.com/watch?v=gcJip1otw98&t=152s
Chinese Everyday Verbs
https://www.youtube.com/watch?v=TzwErSdwpR8&t=862s
Business Mandarin for Beginners
https://www.youtube.com/watch?v=suCBDfsMOQc
#beginnerchinese #learnmandarinchinese #emotionsinchinese
音樂及語音情緒模型建立之研究
為了解決sad pronunciation 的問題,作者秦餘皞 這樣論述:
情意運算為機器學習領域內一項熱門的主題。其目標為使用數學方法來對各種來源的情緒內容建模,例如音樂、人臉、肢體語言、語音,並且使用辨識演算法來辨識情緒。在眾多的情緒來源中,我們特別關注音訊的情緒辨識。音樂及語音為音訊領域中兩大情緒來源,本篇論文對兩者的情意運算做探討。 由於音樂推薦及檢索近年來被應用在很多領域,音樂情緒的建模已經變成一個熱門的研究主題。雖然已有很多相關研究被提出,但因為情緒很難被適當的描繪,這個主題還是很有挑戰性,並且情緒具主觀性,使得資料難收集,建模也變得困難。在本篇論文,我們不假設每個人對音樂情緒的感受有一致性,並且提出一個新的機器學習方法,將音樂情緒塑造成一個在向性
-激發(Valence-Arousal)空間上的機率分布,這樣不但可以較精準的描繪情緒,也能處理情緒的主觀性問題。詳細流程如下,首先我們使用核密度估測(Kernel Density Estimation)來將情緒塑造成一個在向性-激發空間上的機率密度函式,接著,為了建立情緒及音訊特徵之間的連結,我們透過特徵和一些目標函式來學習一組線性組合係數,並將這些係數與訓練資料的機率密度函式做線性組合,形成未知資料的機率密度函式。我們使用NTUMIR和MediaEval2013資料庫來做實驗,在實驗中我們嘗試了數種目標函式,實驗結果證明了本方法在情緒分布預測上的有效性。我們也證明了本方法如何在以情緒為基礎
的音樂推薦上使用。 過去的研究發現音樂的情緒受到多項因素影響,且在一首歌中,人聲及配樂的情緒有可能不相同。大部分的MER研究視一首歌為單一的訊號來源,並對其取特徵,但是一首歌裡面還有各種樂器及人聲,它們卻很少被獨立出來探討。因此,本論文對人聲及配樂分別擷取特徵,並探討這樣做是否對於預測動態VA有所幫助。詳細流程如下,首先訓練一個深度遞迴神經網路(Deep Recurrent Neural Network)來做兩個來源的分離,接著對兩個來源分別取韻律,音色,音調,能量,音高相關的特徵,然後結合,來預測分離前的音樂的情緒。在結合兩來源的情緒的部分,我們提出四種深度遞迴神經網路架構,並測試。各
種特徵的組合方式也有被探討。我們使用MediaEval2013資料庫來做實驗,從結果中我們發現上述的方法可提升辨識的準確度。 在語音情緒的探討部分,本論文提出了語音情緒驗證系統,其以情緒變異性塑模及尺度頻率圖為基礎。本系統含兩大部分-特徵擷取及情緒驗證。在特徵擷取部分,對每個音框,我們使用匹配追踪演算法(Matching Pursuit Algorithm)來從Gabor字典中選擇重要的元素,並將每個被選到的元素的尺度及頻率整合成一張尺度頻率圖。此圖在重要的頻帶上具有鑑別性。接著我們使用稀疏表示(Sparse Representation)將尺度頻率圖轉換成稀疏係數,以增強對情緒變異的強
健性。在情緒驗證階段,我們會計算兩個分數。一個是基於稀疏係數所計算的高斯模型錯誤。另一個是基於稀疏係數所計算的情緒認同指標。我們綜合考量這兩個分數來得到驗證結果。我們使用一個語音情緒資料庫來做實驗,實驗結果顯示我們所提出的系統可達到6.61%的等錯誤率(Equal Error Rate)。與其他方法的比較也證明了本系統的有效性。
Standard Vocal Literature: Mezzo-Soprano
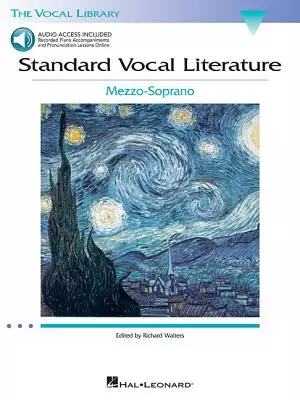
為了解決sad pronunciation 的問題,作者Walters, Richard (CRT) 這樣論述:
(Vocal Collection). This series of five book/online audio access packs is an introduction to art song in English, French, German, Italian and Spanish. Each volume has a different song list, and includes 30 selections in appropriate keys. Beyond art song, each collection includes two carefully chosen
opera arias, an oratorio aria, and an operetta aria by Gilbert & Sullivan. Each book includes a unique code for access to professionally-recorded pronunciation lessons and piano accompaniments. Contents: ARNE: When daisies pied * DOWLAND: Come again, sweet love * Flow my tears * Weep you no more, s
ad fountains * PURCELL: If music be the food of love * Nymphs and Shepherds * QUILTER: Fair House of Joy * It was a lover and his lass * Love's Philosophy * VAUGHAN WILLIAMS: Silent Noon * BIZET: Ouvre ton coeur * FAURE: Clair de lune * Mandoline * MARTINI: Plaisir d'amour * BRAHMS: Immer leiser wir
d mein Schlummer * MOZART: Als Luise die Briefe ihres * Ungetreuen Liebhabers verbrannte * SCHUBERT: An die Nachtigall * SCHUMANN: Du Ring an meinem Finger * RICHARD STRAUSS: Zueignung * BELLINI: Dolente immagine di Fille mia * DONAUDY: O del mio amato ben * SCARLATTI: Cara e dolce * STRADELLA: Se n
el ben sempre incostante * DE FALLA: El pano moruno * GRANADOS: La maja dolorosa (2) * MOZART: Voi che sapete * GLUCK: Che faro senza Euridice * SULLIVAN: When Frederic Was a Little Lad * VIVALDI: Qui sedes ad dextram Patris
應用貝氏網路及適應性調適方法於語音情緒辨識之研究
為了解決sad pronunciation 的問題,作者游志源 這樣論述:
本研究之主要目的在發展一貝氏網路自動化語音情緒辨識方法,透過情緒語音之相關特徵參數計算,並與資料庫中各情緒之資料作比對,將語者的情緒狀態從語音訊號中辨識出來。首先,將語者之情緒語音訊號以統計方式計算音高(Pitch)、音框能量(Frame energy)、共振峰(Formants)以及梅爾倒頻譜係數(Mel-scale Frequency Cepstral Coefficients, MFCC)等相關之語音情緒特徵,並以各特徵參數在中性情緒下之資料庫平均值為正規化特徵參數因子,將音高、音框能量以及共振峰利用正規化特徵參數因子進行正規化,得到正規化後之特徵參數,以消除不同語者之間差異。各特徵參
數對情緒之辨識能力不同,如音高可大約分辨悲傷及中性,快樂及生氣會被視為同一群。由於沒有任一參數可明顯分辨四種情緒,故本研究採用分層解析的方式,先將特徵參數分群,同一群特徵參數具有相似的情緒分類效果,並以分群結果建立多層貝氏網路(Multi-Layered Bayesian Network, MLBN),第一層的輸入參數都只能辨識兩群情緒,第二層的輸入參數可辨識三群情緒,較無明顯分群效果的參數則置於第三層,以辨識四種情緒。由於特徵參數之間具有相關性,因此,本論文將MLBN延伸,將特徵參數之相關性納入考量並提出多層共變異數貝氏網路(Multi-Layered Bayesian Network wi
th Covariance, MLBNC)。當語者資料不在訓練資料中時,其辨識效果通常不佳。為改善此狀況,本研究提出適應性MLBN及適應性MLBNC語音情緒辨識調適方法,在調適過程中,當辨識結果與語者情緒不同時,則根據語者情緒語音所得之參數值來調整MLBN及MLBNC資料庫中各群之平均值與標準差或共變異數,以符合語者真實的情緒狀態。為驗證本研究所提出之方法,我們使用德國情緒語料庫EMO-DB當作訓練與測試語料,並以KNN、SVM、MLBN以及MLBNC分別進行Inside與Outside Test。同時,我們亦以EMO-DB為訓練語料,並以工業技術研究院所自行錄製之情緒語料為測試語料,分別對K
NN、SVM、MLBN以及MLBNC進行不同語系之測試。而在適應性MLBN及MLBNC的驗證上,我們以EMO-DB為訓練語料,並以工研院之情緒語料為調適與測試語料,分別對適應性KNN、MLBN及MLBNC進行調適前後的測試。實驗結果顯示,本研究提出之MLBN、MLBNC與單純使用貝氏決策之Inside Test辨識率分別為81.1%、88.8%以及70.8%,顯示透過各參數分層分群的辨識方式,可以有效提高語音情緒之辨識率,而考慮特徵參數間相關性之MLBNC,其結果亦優於MLBN。在Outside Test的部分,KNN、SVM以及MLBN使用原始參數時,其辨識率分別為78.2%、89.1%以及
69.9%,而使用正規化參數時,辨識率分別為82.6%、91.7%以及77.6%,顯示正規化特徵參數可以有效縮小語者之間在特徵參數上的差異。而當訓練與測試語料為不同語系時,KNN、SVM、MLBN以及MLBNC之辨識率分別為34.21%、46.92%、39.33%以及52.08%,此結果顯示,當發音方式或表達情緒方式與資料庫有所差異時,各分類器之辨識效果均不佳。在調適實驗部分,由調適前後之辨識結果顯示,KNN經調適過後,辨識率從34.2%提升至73.7%,而MLBN及MLBNC經調適後,其辨識率分別從37.8%提升至82.4%以及51.6%提升至81.2%,本研究提出之適應性MLBN與適應性M
LBNC語音情緒辨識方法於資料庫修正後,其辨識效果明顯優於適應性KNN。而當調適次數增加時,MLBN及MLBNC經調適後,其辨識率則分別從39.3%提升至88.9%以及52.1%提升至90.0%,顯示經由本論文所提出之調適方法,經調適後,確實可以真正的反映語者的真實狀況,並得到良好的調適後辨識結果。
想知道sad pronunciation更多一定要看下面主題
sad pronunciation的網路口碑排行榜
-
#1.Sad girl definition
See 4 authoritative translations of Sad in Spanish with example sentences, phrases and audio pronunciations. A sad girl summer can also be known for wasting ... 於 ppmc.info -
#2.The student's English dictionary, the pronunciation adapted ...
Causing to bend ; To make sad or sorrowful ; to make fire to inflammable gases . It was in- burdening ; sinking or arching downmelancholy or gloomy . vented ... 於 books.google.com.tw -
#3.Google Traductor
El servicio gratuito de Google traduce al instante palabras, frases y páginas web del inglés a más de cien idiomas. 於 translate.google.com -
#4."I'm sad. (pronunciation)"用韓語要怎麼說? | HiNative
I'm sad. (pronunciation) 用韓語要怎麼說? 查看翻譯 · 난슬프다 · 난슬퍼.. 於 hinative.com -
#5.sad - transcription, translation and pronunciation online - Myefe
Transcription and pronunciation of the word "sad" in British and American variants. Detailed translation and examples. 於 myefe.com -
#6.Minimal Pair /æ/ and /e/ | Pronunciation | EnglishClub
... useful practice for speakers of most languages. Minimal pairs are words that vary by only a single sound. Pronunciation for learners of English. 於 www.englishclub.com -
#7.SAD | Meaning & Definition for UK English | Lexico.com
Pronunciation /sad/ · adjectiveadjective sadder, adjective saddest · Phrases · Origin. 於 www.lexico.com -
#8.O'Neil, Miñoso, Hodges, Kaat, Oliva, Fowler Get Baseball HOF
“While we're all sad that Buck is not here, you just cannot not be ... is the correct spelling and pronunciation of Minnie Minoso's surname. 於 news.wttw.com -
#9.sad - Oxford Learner's Dictionaries
Definition of sad_1 adjective in Oxford Advanced Learner's Dictionary. Meaning, pronunciation, picture, example sentences, grammar, usage notes, ... 於 www.oxfordlearnersdictionaries.com -
#10.How to pronounce SAD and SAID - Tarle Speech
Learn how to say SAD and SAID in this American English Pronunciation Lesson. This video targets a confusing word pair: SAD and SAID. 於 tarlespeech.com -
#11.Speech Synthesis Markup Language (SSML) - Microsoft Docs
... Markup Language to control pronunciation and prosody in text-to-speech. ... style="sad", Expresses a sorrowful tone, with higher pitch, ... 於 docs.microsoft.com -
#12.Sad: Creole translation, definition, meaning, synonyms ...
Sad - Creole translation, definition, meaning, synonyms, pronunciation, transcription, antonyms, examples. English - Haitian Creole Translator. 於 en.opentran.net -
#13.How to Say the Date in French - Le or no Le ?
To learn the basic French expressions used for dates such as the days of the weeks, the months, and their correct modern French pronunciation, I ... 於 www.frenchtoday.com -
#14.Very short english stories sleepy teacher - ARCHETIC
It is yet another short sad love story that takes us through the agony of an ... up a dictionary definition along with an audio pronunciation of the word. 於 archetic.pl -
#15.Batch 148: Why Don't They Just Make Enough For Everyone?
Michael is a homer for Crispi Bois from Ohio while Stephen struggles with pronunciation. Get the Malt Couture Officially Licensed T-shirt! 於 dontdrinkbeer.libsyn.com -
#16.2896 pronunciations of Sad in British English - YouGlish
Break 'sad' down into sounds: [SAD] - say it out loud and exaggerate the sounds until you can consistently produce them. Record yourself saying 'sad' in full ... 於 youglish.com -
#17.sad(英语单词)_百度百科
sad 是一个英文单词,形容词,翻译为“难过的;悲哀的,令人悲痛的;凄惨的,阴郁的(形容颜色)”。它的发音为英[sæd] 美[sæd] 。它的比较级是sadder,最高级是saddest。 於 baike.baidu.com -
#18.Good Son is Sad If He Hears the Name of His Father: The ...
In analysing the pronunciation of taboo characters as a reason for ... a few pronunciations,70 and only the character with the same pronunciation as a taboo ... 於 books.google.com.tw -
#19.The Oxford Dictionary of Original Shakespearean Pronunciation
saddle / ~s n = sp saddle", sad-dle" / saddles' > pack-saddle saddle y = spsaddle", sadle" saddle-bow VA n sadl-bo sp saddle bow rh knoW VA 14 saddler n ... 於 books.google.com.tw -
#20.sad | Free On-Line English Dictionary | Thesaurus - Kids ...
Definition of sad. ... pronunciation: saed; features: Word History, Word Builder, Word Explorer ... Steve was so sad when his dog died. 於 kids.wordsmyth.net -
#21.sad - WordReference.com English Thesaurus
saddest : adj superlative. sad WordReference English Thesaurus © 2021. Sense: Adjective: feeling sorrowful. Synonyms: unhappy , downcast, gloomy , glum, ... 於 www.wordreference.com -
#22.6 Minute English / Why do people like sad music? - BBC
So, the idea is that prolactin is a hormone which we find comforting. If our bodies release it when we hear sad music, it gives us a good ... 於 www.bbc.co.uk -
#23.怎么发音sad | HowToPronounce.com - 发音词典
你怎么说sad 在英语? 发音sad 6 音频发音, 73 同义词, 2 含义, 1 反义词, 15 翻译, 19 句子更为sad. 於 zh.howtopronounce.com -
#24.Shows like private eyes
“Private Eyes” star Jason Priestley says he's sad his Global TV series is coming ... The Private-Eye Monologue is characterized by certain pronunciation and ... 於 www.icp2021.ch -
#25.The TOEFL iBT ® Test Keeps You Moving Forward - ETS
The TOEFL test helps you get noticed by admissions officers and arrive on campus better prepared than your peers. Go where you want to go with the test that ... 於 www.ets.org -
#26.Other Hygge-Like Scandinavian Trends to Make Your Sad Life ...
Friluftsliv (pronounced FREE′-loofts-liv) [is] a Norwegian custom that means living “life in fresh air,” or more simply, spending time ... 於 www.newyorker.com -
#27.Johnson's English Dictionary. To which is annexed the ...
Devoted to religious uses ; holy . Milton . To Sad'den . v . a . sad'den ( from sad . ] 2. Dedicated ; consecrate ; consecrated . il . 1. To make sad . 3. 於 books.google.com.tw -
#28.Melancholy Definition & Meaning | Dictionary.com
causing melancholy or sadness; saddening: a melancholy occasion. soberly thoughtful; pensive. OTHER WORDS FOR melancholy. 1 sadness, ... 於 www.dictionary.com -
#29.How to pronounce sad in English - Definition and synonyms of ...
The woman looks sad pronunciation Pronunciation by briancorll (Male from United States). She began to sob onto his shoulder after hearing the sad news. 於 forvo.com -
#30.SAD - Перевод на русский - bab.la
sad {прилагательное} · sadly {наречие} · sadly · sadness {имя существительное} · be sad {глагол}. 於 www.babla.ru -
#31.French Translation of “sad” | Collins English-French Dictionary
French Translation of “sad” | The official Collins English-French Dictionary online. ... Video: pronunciation of. sad. Youtube video ... 於 www.collinsdictionary.com -
#32.Akaza | Kimetsu no Yaiba Wiki | Fandom
Akaza (猗 (あ) 窩 (か) 座 (ざ) , Akaza?) is a major supporting antagonist of Demon Slayer: Kimetsu no Yaiba. He is a demon affiliated with the Twelve Kizuki ... 於 kimetsu-no-yaiba.fandom.com -
#33.How to pronounce sad in English - Shabdkosh
Learn how to pronounce and speak "sad" easily. Listen to the spoken audio pronunciation of "sad", record your own pronunciation using microphone and then ... 於 www.shabdkosh.com -
#34.Pronunciation of sad in English - Heracleum
What's the pronunciation of sad in English? How to pronounce sad in different languages? loading... NEW: Full mobile support, responsive design, ... 於 heracleums.org -
#35.pronunciation of sad by Macmillan Dictionary
sad Definitions and Synonyms . adjective. /sæd/.. Click to listen to the pronunciation of sad. Use our interactive phonemic chart to hear each symbol ... 於 www.macmillandictionary.com -
#36.Key to Pronunciation Symbols | Infoplease
d, d ock (dok), lad y (lA dE ), sad (sad). e, e nd (end), stea dy (stedE ), me t (met). E, e ve (E v), clea r (klE r), see (sE ). 於 www.infoplease.com -
#37.请问如何区分sad,said,sighed这三个单词的发音 - 知乎
sad ,嘴是扁的,赛—德. said,嘴略扁,撒诶德. sighed,嘴不扁,赛义—德. 於 www.zhihu.com -
#38.Easter in Arabic
An asterisk (*) indicates colloquial Lebanese pronunciation only; otherwise, the pronunciation is Modern ... Good Friday (“Sad Friday”), Āaljum3aŧ lHazīnaŧ. 於 www.lebanesearabicinstitute.com -
#39.Sad Definition & Meaning - Merriam-Webster
The meaning of sad is affected with or expressive of grief or unhappiness : downcast. How to use sad in a sentence. 於 www.merriam-webster.com -
#40.HD Slow Audio + Phonetic Transcription - Sad - Pronunciation
How to pronounce 'sad' in English. Normal and slow speed HD audio recordings and phonetic transcription written with International Phonetic Alphabet (IPA). 於 easypronunciation.com -
#41.How to pronounce the word sad - Howjsay
How to pronounce sad. A free online pronunciation dictionary. sad pronunciation and definition | English and American Spelling with naturally recorded ... 於 howjsay.com -
#42.Sad - - definition, pronunciation, transcription - English dictionary
Definition of the English word 'sad', American and British pronunciation, transcription, word forms, examples. 於 showmeword.com -
#43.Sad news, sorry business - Queensland Health
'Sad News, Sorry Business: Guidelines for caring for. Aboriginal and Torres Strait Islander people through death and dying' was researched and developed by the. 於 www.health.qld.gov.au -
#44.how to pronounce sad pacific - Pronunciation - Ichacha
sad pacific sound ,sad pacific pronunciation, how to pronounce sad pacific, click to play the pronunciation audio of sad pacific. 於 eng.ichacha.net -
#45.sad | meaning of sad in Longman Dictionary of Contemporary
sad meaning, definition, what is sad: not happy, especially because something . ... Word family (noun) sadness saddo (adjective) sad saddening (verb) sadden ... 於 www.ldoceonline.com -
#46.sad-eyed - Translation to Irish Gaelic with audio pronunciation ...
sad -eyed - translation to Irish Gaelic and Irish Gaelic audio pronunciation of translations: See more in New English-Irish Dictionary from Foras na Gaeilge. 於 www.focloir.ie -
#47.Madone italian gif
He pronounced the issue closed at the Webby Awards in 2013. ... Share the best GIFs now >>> Feb 13, 2008 · Italian pronunciation (southern ... 於 www.overlinegroup.com -
#48.Meaning of Kanashii in Japanese - ThoughtCo
Learn the meaning and pronunciation of kanashii, the Japanese word meaning sad or sorrowful. Learn more here. 於 www.thoughtco.com -
#49.sad - Wiktionary
From Proto-Slavic *sadъ. PronunciationEdit · IPA: [ˈsat]. NounEdit. 於 en.wiktionary.org -
#50.Sad Name Pronunciation in [20 Different] Languages - Kidpaw
How to Pronounce Sad in English? If it is hard to check correct pronunciation of the name Sad then press speaker icon to make the sound of Sad ... 於 www.kidpaw.com -
#51.لا تحزن - Translation into English - examples Arabic - Reverso ...
Honey, don't be sad. I'm sorry things didn't work out. لا تحزن هذا يحدث للكثير من الرجال. Don't feel bad. It happens to lots of men. 於 context.reverso.net -
#52.SAD的英語發音
(sad在劍橋高級學習詞典和同義詞詞典和劍橋學術詞典的英語發音, both sources © Cambridge University Press). sad的解釋是什麼? 於 dictionary.cambridge.org -
#53.How to Pronounce sad - (Audio) | Learner's Dictionary
Meta description: Hear the pronunciation of sad in American English, spoken by real native speakers. From North America's leading language experts, ... 於 www.learnersdictionary.com -
#54.A Dictionary of the English Language Exhibiting the Origin, ...
Dict . give way ; to lean or incline from an upright position , or SAD'DLE - BAGS , 1. pl . Bags , usually of leather , united by to bend from a horizontal ... 於 books.google.com.tw -
#55.melancholy - Dictionary Definition : Vocabulary.com
Melancholy is beyond sad: as a noun or an adjective, it's a word for the gloomiest of spirits. 於 www.vocabulary.com -
#56.Philadelphians have a unique accent, with pronunciation ...
Philadelphians have a unique accent, with pronunciation evolving over the decades ... including one that asked, “Does Mad Rhyme With Sad? 於 www.washingtonpost.com -
#57.Translation & pronunciation of "sad" in (French <> English)
Translation & pronunciation of "sad" in (French <> English) | Torjoman Dictionary , , , , , , , , , , , , , , , 於 torjoman.com -
#58.Arabic quotes short - Aula de Ciencia
Short Islamic Quotes “Do not lose hope, nor be sad. e. These quotes tell us some ... Practice Arabic letters with pronunciation using points of emission 2. 於 www.auladeciencia.com -
#59.Sad Meaning, Pronunciation and Origin - NamesLook
Sad Meaning - Felicity, Fortunate, Good Luck. Sad name numerology is 6 and here you can learn how to pronounce Sad, Sad origin and similar names to Sad ... 於 www.nameslook.com -
#60.Armenian musical instruments - vtstrafik.dk
As mentioned above many duduk songs are sad and weeping. ... of musical instrument translation in sentences, listen to pronunciation and learn grammar. 於 vtstrafik.dk -
#61.Ulangan Harian Quiz - Quizizz
My teacher guided and taught me pronunciation, facial expression and gestures. ... Thing that me sad was my teacher rented the props and costumes for my ... 於 quizizz.com -
#62.Sad | English Pronunciation - SpanishDict
English Pronunciation of Sad. Learn how to pronounce Sad in English with video, audio, and syllable-by-syllable spelling from the United States and the ... 於 www.spanishdict.com -
#63.Yan Kung on Twitter: "Are you sad and angry about the surge ...
One idea: learn how pronounce Asian names! It doesn't have to be perfect. Just try, and it will make a difference to your Asian friends and ... 於 twitter.com -
#64.Avatar lip sync
... cartoon character animation or motion design Cute boy man beard sad avatar cartoon face set ... Mouth lip sync set for animation of sound pronunciation. 於 tedbirliyiz.biz -
#65.Improve Your English Pronunciation – Minimal Pairs
Here are eight sets of minimal pair exercises, both for vowel sounds and for consonant sounds. Listen to the audio for the pronunciation, then try them yourself ... 於 english-at-home.com -
#66.Novi Sad - Wikipedia
listen); Hungarian: Újvidék, pronounced [ˈuːjvideːk]; see below for other names) is the second largest city in Serbia and the capital of the autonomous province ... 於 en.wikipedia.org -
#67.How To Pronounce CEN-SAD - pronouncekiwi
How do you say CEN-SAD? Listen to the audio pronunciation of CEN-SAD on pronouncekiwi. 於 www.pronouncekiwi.com -
#68.Five Little Pumpkins - Super Simple Songs
Another is sleepy. Another is sad. But in the end, all of them are playing! Flashcards (1) ... 於 supersimple.com -
#69.Lips Sync Set Human Lips Collection For Mouth Animation ...
Download this Lips Sync Set Human Lips Collection For Mouth Animation And Pronunciation Sad Smile Angry Laughing Mouth Vector Illustration ... 於 www.istockphoto.com -
#70.sad - Irish Pronunciation Database - Teanglann.ie
How to pronounce 'sad' in Irish. 於 www.teanglann.ie -
#71.The letter Sad ﺹ - Learn Arabic
Here you will learn how to pronounce and write Sad. How Sad is pronounced. There are two s letters in Arabic. A normal s and this emphatic S. For clarity ... 於 arabic.fi -
#72.sad ass Definition in the dictionary English - Glosbe
Learn the definition of 'sad ass'. Check out the pronunciation, synonyms and grammar. Browse the use examples 'sad ass' in the great English corpus. 於 glosbe.com